Discovery and Implementation of Fast, Accurate and Transferable Many-Body Interatomic Potentials
Adarsh Balasubramanian
2019.
Four Generations of High-Dimensional Neural Network Potentials
Jörg Behler
Chemical Reviews, 2021.
DOI: 10.1021/acs.chemrev.0c00868
Dynamical Processes in the Condensed Phase: Methods and Models
Matthew Ralph Carbone
2021.
Machine Learning and the Physical Sciences
Giuseppe Carleo, Ignacio Cirac, Kyle Cranmer, Laurent Daudet, Maria Schuld, Naftali Tishby, Leslie Vogt-Maranto, Lenka Zdeborova
Reviews of Modern Physics, 2019, 91 (4), 045002.
DOI: 10.1103/RevModPhys.91.045002
Autonomous Discovery in the Chemical Sciences Part I: Progress
Connor W. Coley, Natalie S. Eyke, Klavs F. Jensen
Angewandte Chemie-International Edition, 2020, 59 (51), 22858–22893.
DOI: 10.1002/anie.201909987
Designing Models Using Machine Learning: One-Body Reduced Density Matrices and Spectra
Andrea Costamagna
2020.
Interfacial Potentials in Ion Solvation
Carrie Conor Doyle
2020.
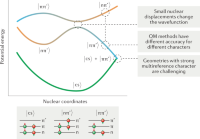
Molecular Excited States through a Machine Learning Lens
Pavlo O. Dral, Mario Barbatti
Nature Reviews Chemistry, 2021, 5 (6), 388–405.
DOI: 10.1038/s41570-021-00278-1
Characterizing Magnetic Skyrmions at Their Fundamental Length and Time Scales
Peter Fischer, Sujoy Roy
Magnetic Skyrmions and Their Applications, 2021, 55–97.
Unsupervised Learning Methods for Molecular Simulation Data
Aldo Glielmo, Brooke E. Husic, Alex Rodriguez, Cecilia Clementi, Frank Noé, Alessandro Laio
Chemical Reviews, 2021.
DOI: 10.1021/acs.chemrev.0c01195
The Structure and Dynamics of Materials Using Machine Learning
Mário Rui Gonçalves Marques
2020.
Machine-Learning-Assisted Modeling
Sarah Greenstreet
Physics Today, 2021, 74 (7), 42–47.
DOI: 10.1063/PT.3.4794
Atomic-Scale Representation and Statistical Learning of Tensorial Properties
Andrea Grisafi, David M. Wilkins, Michael J. Willatt, Michele Ceriotti
Machine Learning in Chemistry: Data-Driven Algorithms, Learning Systems, and Predictions, 2019, 1–21.
Adaptive Iron-Based Magnetic Nanomaterials of High Performance for Biomedical Applications
Ning Gu, Zuoheng Zhang, Yan Li
Nano Research, 2021.
DOI: 10.1007/s12274-021-3546-1
Deep Learning for Large-Scale Molecular Dynamics and High-Dimensional Partial Differential Equations
Jiequn Han
2018.
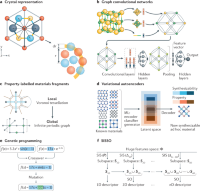
Machine Learning for Alloys
Gus L. W. Hart, Tim Mueller, Cormac Toher, Stefano Curtarolo
Nature Reviews Materials, 2021.
DOI: 10.1038/s41578-021-00340-w
Characterizing Performance Improvement of GPUs
Dodi Heryadi, Scott Hampton
Proceedings of the Practice and Experience in Advanced Research Computing on Rise of the Machines (Learning), 2019, 1–5.
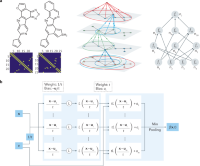
Physics-Informed Machine Learning
George Em Karniadakis, Ioannis G. Kevrekidis, Lu Lu, Paris Perdikaris, Sifan Wang, Liu Yang
Nature Reviews Physics, 2021, 3 (6), 422–440.
DOI: 10.1038/s42254-021-00314-5
Neural Network Potentials: A Concise Overview of Methods
Emir Kocer, TW Tsz Wai Ko, Jörg Behler, J Behler
arxiv.org, 2021.
First-Principles Study on the Structural and Thermal Properties of Molecular Crystals and Liquids
Hsin-Yu Ko
2019.
FMO Interfaced with Molecular Dynamics Simulation
Yuto Komeiji, Takeshi Ishikawa
Recent Advances of the Fragment Molecular Orbital Method, 2021, 373–389.
Classical Molecular Dynamics Using Neural Network Representations of Potential Energy Surfaces
Andreas Godø Lefdalsnes
2019.
Modeling Electrified Metal/Water Interfaces from Ab Initio Molecular Dynamics: Structure and Helmholtz Capacitance
Jia-Bo Le, Jun Cheng
Current Opinion in Electrochemistry, 2021, 27, 100693.
DOI: 10/ghtqnk
Molecular Dynamics Study of Charged Nanomaterials: Electrostatics and Self-Assembly
Yaohua Li
2021.
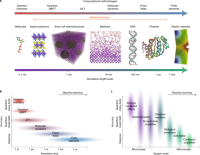
Discovering and Understanding Materials through Computation
Steven G. Louie, Yang-Hao Chan, Felipe H. da Jornada, Zhenglu Li, Diana Y. Qiu
Nature Materials, 2021, 20 (6), 728–735.
DOI: 10.1038/s41563-021-01015-1
Future Directions of Chemical Theory and Computation
Yuyuan Lu, Geng Deng, Zhigang Shuai
Pure and Applied Chemistry, 2021.
DOI: 10.1515/pac-2020-1006
Integrating Machine Learning into Protein-Ligand Scoring Function Development
Jianing Lu
2020.
Development of a Machine Learning Potential for Nucleotides in Water
Riccardo Martina
, 57.
Machine Learning for Chemical Reactions
Markus Meuwly
Chemical Reviews, 2021.
DOI: 10.1021/acs.chemrev.1c00033
Strategies for the Construction of Machine-Learning Potentials for Accurate and Efficient Atomic-Scale Simulations
April M. Miksch, Tobias Morawietz, Johannes Kaestner, Alexander Urban, Nongnuch Artrith
Machine Learning-Science and Technology, 2021, 2 (3), 031001.
DOI: 10.1088/2632-2153/abfd96
Membrane Models for Molecular Simulations of Peripheral Membrane Proteins
Mahmoud Moqadam, Thibault Tubiana, Emmanuel E. Moutoussamy, Nathalie Reuter
Advances in Physics-X, 2021, 6 (1), 1932589.
DOI: 10.1080/23746149.2021.1932589
Machine Learning-Accelerated Quantum Mechanics-Based Atomistic Simulations for Industrial Applications
Tobias Morawietz, Nongnuch Artrith
Journal of Computer-Aided Molecular Design, 2021, 35 (4), 557–586.
DOI: 10.1007/s10822-020-00346-6
Physics-Inspired Structural Representations for Molecules and Materials
Felix Musil, Andrea Grisafi, Albert P. Bartók, Christoph Ortner, Gábor Csányi, Michele Ceriotti
Chemical Reviews, 2021.
DOI: 10.1021/acs.chemrev.1c00021
Computational Predictions of the Thermal Conductivity of Solids and Liquids
Marcello Puligheddu
2020.
RANDOM PHASE APPROXIMATION AND BEYOND: FROM THEORY TO REALISTIC MATERIALS
Dario Rocca
2020.
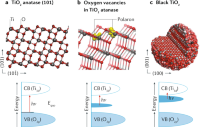
Theoretical Insights into the Surface Physics and Chemistry of Redox-Active Oxides
Roger Rousseau, Vassiliki-Alexandra Glezakou, Annabella Selloni
Nature Reviews Materials, 2020, 5 (6), 460–475.
DOI: 10.1038/s41578-020-0198-9
Construction of Machine Learned Force Fields with Quantum Chemical Accuracy: Applications and Chemical Insights
Huziel E. Sauceda, Stefan Chmiela, Igor Poltavsky, Klaus-Robert Müller, Alexandre Tkatchenko
Machine Learning Meets Quantum Physics, 2020, 277–307.
Interatomic Potential for Li-C Systems from Cluster Expansion to Artificial Neural Network Techniques
Yusuf Shaidu
2020.
Modelling Bulk Electrolytes and Electrolyte Interfaces with Atomistic Machine Learning
Yunqi Shao, Lisanne Knijff, Florian M. Dietrich, Kersti Hermansson, Chao Zhang
Batteries \& Supercaps, 2021, 4 (4), 585–595.
DOI: 10.1002/batt.202000262
Neural Network for the Prediction of Force Differences between an Amino Acid in Solution and Vacuum
Gopal Narayan Srivastava
2020.
Machine Learning Force Fields
Oliver T. Unke, Stefan Chmiela, Huziel E. Sauceda, Michael Gastegger, Igor Poltavsky, Kristof T. Schütt, Alexandre Tkatchenko, Klaus-Robert Müller
Chemical Reviews, 2021.
DOI: 10.1021/acs.chemrev.0c01111
Challenges for Machine Learning Force Fields in Reproducing Potential Energy Surfaces of Flexible Molecules
Valentin Vassilev-Galindo, Gregory Fonseca, Igor Poltavsky, Alexandre Tkatchenko
Journal of Chemical Physics, 2021, 154 (9), 094119.
DOI: 10.1063/5.0038516
Force Field Development and Nanoreactor Chemistry
Lee-Ping Wang
Computational Approaches for Chemistry Under Extreme Conditions, 2019, 127–159.
Investigations of Water/Oxide Interfaces by Molecular Dynamics Simulations
Ruiyu Wang, Michael L. Klein, Vincenzo Carnevale, Eric Borguet
Wiley Interdisciplinary Reviews-Computational Molecular Science, 2021, e1537.
DOI: 10.1002/wcms.1537
Physics-Guided Deep Learning for Dynamical Systems: A Survey
Rui Wang
2021.
Integrating Machine Learning with Physics-Based Modeling
E Weinan, Jiequn Han, Zhang Linfeng
2020.
Machine Learning and Computational Mathematics
E. Weinan
Communications in Computational Physics, 2020, 28 (5), 1639–1670.
DOI: 10.4208/cicp.OA-2020-0185
Machine Learning for Electronically Excited States of Molecules
Julia Westermayr, Philipp Marquetand
Chemical Reviews, 2020.
DOI: 10.1021/acs.chemrev.0c00749
Integrating Physics-Based Modeling with Machine Learning: A Survey
J Willard, X Jia, S Xu, M Steinbach, V Kumar
arxiv.org, 2021.
Deep Learning Methods for the Design and Understanding of Solid Materials
Tian Xie
2020.
Perspective on Computational Reaction Prediction Using Machine Learning Methods in Heterogeneous Catalysis
Jiayan Xu, Xiao-Ming Cao, P. Hu
Physical Chemistry Chemical Physics, 2021, 23 (19), 11155–11179.
DOI: 10.1039/d1cp01349a
Recent Progress on Multiscale Modeling of Electrochemistry
Xiao‐Hui Yang, Yong‐Bin Zhuang, Jia‐Xin Zhu, Jia‐Bo Le, Jun Cheng
WIREs Computational Molecular Science, 2021.
DOI: 10.1002/wcms.1559
Machine Learning of Coarse-Grained Models for Organic Molecules and Polymers: Progress, Opportunities, and Challenges
Huilin Ye, Weikang Xian, Ying Li
ACS omega, 2021, 6 (3), 1758–1772.
DOI: 10.1021/acsomega.0c05321
Machine Learning for Multi-Scale Molecular Modeling: Theories, Algorithms, and Applications
L Zhang
2020.
Global Optimization of Chemical Cluster Structures: Methods, Applications, and Challenges
Jun Zhang, Vassiliki-Alexandra Glezakou
International Journal of Quantum Chemistry, 2021, 121 (7), e26553.
DOI: 10.1002/qua.26553
Non-Contact Ultrasound
Xiang Zhang
2019.