The following publications have used the DeePMD-kit software. Publications that only mentioned the DeePMD-kit will not be included below.
We encourage explicitly mentioning DeePMD-kit with proper citations in your publications, so we can more easily find and list these publications.
Last update date: Nov 28, 2023
2024
Ultrafast switching dynamics of the ferroelectric order in stacking- engineered ferroelectrics
Ri He, Bingwen Zhang, Hua Wang, Lei Li, Ping Tang, Gerrit Bauer, Zhicheng Zhong
Acta Materialia, 2024, 262, 119416.
DOI: 10.1016/j.actamat.2023.119416
Unraveling pyrolysis mechanisms of lignin dimer model compounds: Neural network-based molecular dynamics simulation investigations
Zhe Shang, Hui Li
Fuel, 2024, 357, 129909.
DOI: 10.1016/j.fuel.2023.129909
Mobile dislocation mediated Hall-Petch and inverse Hall-Petch behaviors in nanocrystalline Al-doped boron carbide
Jun Li, Kun Luo, Qi An
Journal of the European Ceramic Society, 2024, 44, 659–667.
DOI: 10.1016/j.jeurceramsoc.2023.09.079
2023
Machine learning interatomic potential for molecular dynamics simulation of the ferroelectric KNbO3 perovskite
Hao-Cheng Thong, XiaoYang Wang, Jian Han, Linfeng Zhang, Bei Li, Ke Wang, Ben Xu
Phys. Rev. B, 2023, 107, 14101.
DOI: 10.1103/PhysRevB.107.014101
Li ion diffusion behavior of Li3OCl solid-state electrolytes with different defect structures: insights from the deep potential model
Zhou Zhang, Zhongyun Ma, Yong Pei
Phys. Chem. Chem. Phys., 2023, 25, 13297–13307.
DOI: 10.1039/d2cp06073f
A deep learning-based potential developed for calcium silicate hydrates with both high accuracy and efficiency
Weihuan Li, Yang Zhou, Li Ding, Pengfei Lv, Yifan Su, Rui Wang, Changwen Miao
Journal of Sustainable Cement-Based Materials, 2023, 12, 1335–1346.
DOI: 10.1080/21650373.2023.2219251
Tuning the lattice thermal conductivity of Sb2Te3 by Cr doping: a deep potential molecular dynamics study
Pan Zhang, Wenkai Liao, Ziyang Zhu, Mi Qin, Zhenhua Zhang, Dan Jin, Yong Liu, Ziyu Wang, Zhihong Lu, Rui Xiong
Phys. Chem. Chem. Phys., 2023, 25, 15422–15432.
DOI: 10.1039/d3cp00999h
A deep learning interatomic potential suitable for simulating radiation damage in bulk tungsten
Chang-Jie Ding, Ya-Wei Lei, Xiao-Yang Wang, Xiao-Lin Li, Xiang-Yan Li, Yan-Ge Zhang, Yi-Chun Xu, Chang-Song Liu, Xue-Bang Wu
Tungsten, 2023.
DOI: 10.1007/s42864-023-00230-4
Deep-learning potentials for proton transport in double-sided graphanol
Siddarth K. Achar, Leonardo Bernasconi, Juan J. Alvarez, J. Karl Johnson
Journal of Materials Research, 2023.
DOI: 10.1557/s43578-023-01141-3
A deep potential molecular dynamics study on the ionic structure and transport properties of NaCl-CaCl2 molten salt
Gegentana, Liu Cui, Leping Zhou, Xiaoze Du
Ionics, 2023, 1–11.
A first-principles machine-learning force field for heterogeneous ice nucleation on microcline feldspar
Pablo M Piaggi, Annabella Selloni, Athanassios Z Panagiotopoulos, Roberto Car, Pablo G Debenedetti
Faraday Discuss., 2023.
DOI: 10.1039/d3fd00100h
Accelerating materials discovery using integrated deep machine learning approaches
Weiyi Xia, Ling Tang, Huaijun Sun, Chao Zhang, Kai-Ming Ho, Gayatri Viswanathan, Kirill Kovnir, Cai-Zhuang Wang
J. Mater. Chem. A, 2023.
DOI: 10.1039/d3ta03771a
Speciation of La3+-Cl- Complexes in Hydrothermal Fluids from Deep Potential Molecular Dynamics
Wei Zhang, Li Zhou, Tinggui Yan, Mohan Chen
J. Phys. Chem. B, 2023, 127, 8926–8937.
DOI: 10.1021/acs.jpcb.3c05428
Neural Network Water Model Based on the MB-Pol Many-Body Potential
Maria Carolina Muniz, Roberto Car, Athanassios Z Panagiotopoulos
J. Phys. Chem. B, 2023, 127, 9165–9171.
DOI: 10.1021/acs.jpcb.3c04629
Understanding the Anomalous Diffusion of Water in Aqueous Electrolytes Using Machine Learned Potentials
Nikhil V S Avula, Michael L Klein, Sundaram Balasubramanian
J. Phys. Chem. Lett., 2023, 14, 9500–9507.
DOI: 10.1021/acs.jpclett.3c02112
Insights into the local structure evolution and thermophysical properties of NaCl-KCl-MgCl2\texte ndashLaCl3 melt driven by machine learning
Jia Zhao, Taixi Feng, Guimin Lu, Jianguo Yu
J. Mater. Chem. A, 2023, 11, 23999–24012.
DOI: 10.1039/d3ta03434h
Constrained Hybrid Monte Carlo Sampling Made Simple for Chemical Reaction Simulations
Bin Jin, Taiping Hu, Kuang Yu, Shenzhen Xu
J. Chem. Theory Comput., 2023, 19, 7343–7357.
DOI: 10.1021/acs.jctc.3c00571
Water dissociation at the water-rutile TiO 2 (110) interface from ab~initio-based deep neural network simulations
Bo Wen, Marcos F Calegari Andrade, Li-Min Liu, Annabella Selloni
Proc. Natl. Acad. Sci. U. S. A., 2023, 120, e2212250120.
DOI: 10.1073/pnas.2212250120
Development and Validation of Versatile Deep Atomistic Potentials for Metal Oxides
Pandu Wisesa, Christopher M Andolina, Wissam A Saidi
J. Phys. Chem. Lett., 2023, 14, 468–475.
DOI: 10.1021/acs.jpclett.2c03445
Machine learning assisted investigation of the barocaloric performance in ammonium iodide
Xiong Xu, Fangbiao Li, Chang Niu, Min Li, Hui Wang
2023, 122.
DOI: 10.1063/5.0131696
Accessing the thermal conductivities of Sb2Te3 and Bi2Te3/Sb2Te3 superlattices by molecular dynamics simulations with a deep neural network potential
Pan Zhang, Mi Qin, Zhenhua Zhang, Dan Jin, Yong Liu, Ziyu Wang, Zhihong Lu, Jing Shi, Rui Xiong
Phys. Chem. Chem. Phys., 2023, 25, 6164–6174.
DOI: 10.1039/d2cp05590b
Predicting Structural Properties of Pure Silica Zeolites Using Deep Neural Network Potentials
Tyler G Sours, Ambarish R Kulkarni
J. Phys. Chem. C. Nanomater. Interfaces, 2023, 127, 1455–1463.
DOI: 10.1021/acs.jpcc.2c08429
Melting of MgSiO3 determined by machine learning potentials
Jie Deng, Haiyang Niu, Junwei Hu, Mingyi Chen, Lars Stixrude
Phys. Rev. B, 2023, 107, 64103.
DOI: 10.1103/PhysRevB.107.064103
Machine learning based implicit solvent model for aqueous-solution alanine dipeptide molecular dynamics simulations
Songyuan Yao, Richard Van, Xiaoliang Pan, Ji Hwan Park, Yuezhi Mao, Jingzhi Pu, Ye Mei, Yihan Shao
RSC Adv., 2023, 13, 4565–4577.
DOI: 10.1039/d2ra08180f
Large-scale atomistic simulation of dislocation core structure in face-centered cubic metal with Deep Potential method
Fenglin Deng, Hongyu Wu, Ri He, Peijun Yang, Zhicheng Zhong
Computational Materials Science, 2023, 218, 111941.
DOI: 10.1016/j.commatsci.2022.111941
Melting conditions and entropies of superionic water ice: Free-energy calculations based on hybrid solid/liquid reference systems
Vitor Fidalgo C\^andido, Filipe Matusalem, Maurice de Koning
J. Chem. Phys., 2023, 158, 64502.
DOI: 10.1063/5.0138987
Size and Quality of Quantum Mechanical Data Set for Training Neural Network Force Fields for Liquid Water
M'arcio S Gomes-Filho, Alberto Torres, Alexandre Reily Rocha, Luana S Pedroza
J. Phys. Chem. B, 2023, 127, 1422–1428.
DOI: 10.1021/acs.jpcb.2c09059
Thermal transport across copper-water interfaces according to deep potential molecular dynamics
Zhiqiang Li, Xiaoyu Tan, Zhiwei Fu, Linhua Liu, Jia-Yue Yang
Phys. Chem. Chem. Phys., 2023, 25, 6746–6756.
DOI: 10.1039/d2cp05530a
Quasiclassical Trajectory Simulation as a Protocol to Build Locally Accurate Machine Learning Potentials
Jintu Zhang, Haotian Zhang, Zhixin Qin, Yu Kang, Xin Hong, Tingjun Hou
J. Chem. Inf. Model., 2023, 63, 1133–1142.
DOI: 10.1021/acs.jcim.2c01497
QD$\pi$: A Quantum Deep Potential Interaction Model for Drug Discovery
Jinzhe Zeng, Yujun Tao, Timothy J Giese, Darrin M York
J. Chem. Theory Comput., 2023, 19, 1261–1275.
DOI: 10.1021/acs.jctc.2c01172
A deep learning approach to predict thermophysical properties of metastable liquid Ti-Ni-Cr-Al alloy
R. L. Xiao, Q. Wang, J. Y. Qin, J. F. Zhao, Y. Ruan, H. P. Wang, H. Li, B. Wei
2023, 133.
DOI: 10.1063/5.0138001
A \textquotedblleftshort blanket\textquotedblright dilemma for a state-of-the-art neural network potential for water: Reproducing experimental properties or the physics of the underlying many-body interactions?
Yaoguang Zhai, Alessandro Caruso, Sigbj\orn L\oland Bore, Zhishang Luo, Francesco Paesani
J. Chem. Phys., 2023, 158, 84111.
DOI: 10.1063/5.0142843
Structure and solidification of the (Fe0.75B0.15Si0.1)100-xTax (x=0-2) melts: Experiment and machine learning
I.V. Sterkhova, L.V. Kamaeva, V.I. Lad'yanov, N.M. Chtchelkatchev
Journal of Physics and Chemistry of Solids, 2023, 174, 111143.
DOI: 10.1016/j.jpcs.2022.111143
Unravelling the dissolution dynamics of silicate minerals by deep learning molecular dynamics simulation: A case of dicalcium silicate
Yunjian Li, Hui Pan, Zongjin Li
Cement and Concrete Research, 2023, 165, 107092.
DOI: 10.1016/j.cemconres.2023.107092
Profiling the off-center atomic displacements in CuCl at finite temperatures with a deep-learning potential
Zhi-Hao Wang, Xuan-Yan Chen, Zhen Zhang, Xie Zhang, Su- Huai Wei
Phys. Rev. Materials, 2023, 7, 34601.
DOI: 10.1103/PhysRevMaterials.7.034601
Liquid-Crystal Structure Inheritance in Machine Learning Potentials for Network-Forming Systems
I. A. Balyakin, R. E. Ryltsev, N. M. Chtchelkatchev
Jetp Lett., 2023, 117, 370–376.
DOI: 10.1134/S0021364023600234
Oxygen Vacancy Diffusion in Rutile TiO2: Insight from Deep Neural Network Potential Simulations
Zhihong Wu, Wen-Jin Yin, Bo Wen, Dongwei Ma, Li-Min Liu
J. Phys. Chem. Lett., 2023, 14, 2208–2214.
DOI: 10.1021/acs.jpclett.2c03827
Nanotwinning-induced pseudoplastic deformation in boron carbide under low temperature
Jun Li, Qi An
International Journal of Mechanical Sciences, 2023, 242, 107998.
DOI: 10.1016/j.ijmecsci.2022.107998
Quantum Informed Machine-Learning Potentials for Molecular Dynamics Simulations of CO2\textquoterights Chemisorption and Diffusion in Mg-MOF-74
Bowen Zheng, Felipe Lopes Oliveira, Rodrigo Neumann Barros Ferreira, Mathias Steiner, Hendrik Hamann, Grace X Gu, Binquan Luan
ACS Nano, 2023, 17, 5579–5587.
DOI: 10.1021/acsnano.2c11102
Modern semiempirical electronic structure methods and machine learning potentials for drug discovery: Conformers, tautomers, and protonation states
Jinzhe Zeng, Yujun Tao, Timothy J Giese, Darrin M York
J. Chem. Phys., 2023, 158, 124110.
DOI: 10.1063/5.0139281
Innate dynamics and identity crisis of a metal surface unveiled by machine learning of atomic environments
Matteo Cioni, Daniela Polino, Daniele Rapetti, Luca Pesce, Massimo Delle Piane, Giovanni M Pavan
J. Chem. Phys., 2023, 158, 124701.
DOI: 10.1063/5.0139010
Prediction on local structure and properties of LiCl-KCl-AlCl3 ternary molten salt with deep learning potential
Min Bu, Taixi Feng, Guimin Lu
Journal of Molecular Liquids, 2023, 375, 120689.
DOI: 10.1016/j.molliq.2022.120689
Atomic structure, stability, and dissociation of dislocations in cadmium telluride
Jun Li, Kun Luo, Qi An
International Journal of Plasticity, 2023, 163, 103552.
DOI: 10.1016/j.ijplas.2023.103552
The highest melting point material: Searched by Bayesian global optimization with deep potential molecular dynamics
Yinan Wang, Bo Wen, Xingjian Jiao, Ya Li, Lei Chen, Yujin Wang, Fu-Zhi Dai
2023, 12, 803–814.
DOI: 10.26599/JAC.2023.9220721
High-Accuracy Neural Network Interatomic Potential for Silicon Nitride
Hui Xu, Zeyuan Li, Zhaofu Zhang, Sheng Liu, Shengnan Shen, Yuzheng Guo
Nanomaterials (Basel)., 2023, 13, 1352.
DOI: 10.3390/nano13081352
A New Spinel Chloride Solid Electrolyte with High Ionic Conductivity and Stability for Na-Ion Batteries
Jiahui Liu, Shuo Wang, Yoshiyuki Kawazoe, Qiang Sun
ACS Materials Lett., 2023, 5, 1009–1017.
DOI: 10.1021/acsmaterialslett.3c00119
Scalable hybrid deep neural networks/polarizable potentials biomolecular simulations including long-range effects
Th'eo Jaffrelot Inizan, Thomas Pl'e, Olivier Adjoua, Pengyu Ren, Hatice G"okcan, Olexandr Isayev, Louis Lagard\`ere, Jean-Philip Piquemal
Chem. Sci., 2023, 14, 5438–5452.
DOI: 10.1039/d2sc04815a
Structural and Composition Evolution of Palladium Catalyst for CO Oxidation under Steady-State Reaction Conditions
Jiawei Wu, Dingming Chen, Jianfu Chen, Haifeng Wang
J. Phys. Chem. C, 2023, 127, 6262–6270.
DOI: 10.1021/acs.jpcc.2c07877
Monitoring the melting behavior of boron nanoparticles using a neural network potential
Xiaoya Chang, Qingzhao Chu, Dongping Chen
Phys. Chem. Chem. Phys., 2023, 25, 12841–12853.
DOI: 10.1039/d3cp00571b
Microstructure and Thermophysical Property Prediction for Chloride Composite Phase Change Materials: A Deep Potential Molecular Dynamics Study
C. Y. Zhao, Y. B. Tao, Y. He
J. Phys. Chem. C, 2023, 127, 6852–6860.
DOI: 10.1021/acs.jpcc.2c08589
Cooperative diffusion in body-centered cubic iron in Earth and super- Earths\textquoteright inner core conditions
Maitrayee Ghosh, Shuai Zhang, Lianming Hu, S X Hu
J. Phys. Condens. Matter, 2023, 35, 154002.
DOI: 10.1088/1361-648X/acba71
Pressure-Induced Stability of Methane Hydrate from Machine Learning Force Field Simulations
Kun Luo, Yidi Shen, Jun Li, Qi An
J. Phys. Chem. C, 2023, 127, 7071–7077.
DOI: 10.1021/acs.jpcc.2c09121
Unraveling the Dynamic Correlations between Transition Metal Migration and the Oxygen Dimer Formation in the Highly Delithiated LixCoO2 Cathode
Taiping Hu, Fu-Zhi Dai, Guobing Zhou, Xiaoxu Wang, Shenzhen Xu
J. Phys. Chem. Lett., 2023, 14, 3677–3684.
DOI: 10.1021/acs.jpclett.3c00506
Predicting thermodynamic stability of magnesium alloys in machine learning
Xi He, Jinde Liu, Chen Yang, Gang Jiang
Computational Materials Science, 2023, 223, 112111.
DOI: 10.1016/j.commatsci.2023.112111
Hydrogen distribution between the Earth's inner and outer core
Liang Yuan, Gerd Steinle-Neumann
Earth and Planetary Science Letters, 2023, 609, 118084.
DOI: 10.1016/j.epsl.2023.118084
Lattice Thermal Conductivity of Monolayer InSe Calculated by Machine Learning Potential
Jinsen Han, Qiyu Zeng, Ke Chen, Xiaoxiang Yu, Jiayu Dai
Nanomaterials (Basel)., 2023, 13, 1576.
DOI: 10.3390/nano13091576
Modeling the high-pressure solid and liquid phases of tin from deep potentials with ab initio accuracy
Tao Chen, Fengbo Yuan, Jianchuan Liu, Huayun Geng, Linfeng Zhang, Han Wang, Mohan Chen
Phys. Rev. Materials, 2023, 7, 53603.
DOI: 10.1103/PhysRevMaterials.7.053603
Estimation of frequency factors for the calculation of kinetic isotope effects from classical and path integral free energy simulations
Timothy J Giese, Darrin M York
J. Chem. Phys., 2023, 158.
DOI: 10.1063/5.0147218
A Deep Potential model for liquid-vapor equilibrium and cavitation rates of water
Ignacio Sanchez-Burgos, Maria Carolina Muniz, Jorge R Espinosa, Athanassios Z Panagiotopoulos
J. Chem. Phys., 2023, 158.
DOI: 10.1063/5.0144500
First-Principles-Based Machine Learning Models for Phase Behavior and Transport Properties of CO2
Reha Mathur, Maria Carolina Muniz, Shuwen Yue, Roberto Car, Athanassios Z Panagiotopoulos
J. Phys. Chem. B, 2023, 127, 4562–4569.
DOI: 10.1021/acs.jpcb.3c00610
Characterizing Structure-Dependent TiS2/Water Interfaces Using Deep-Neural-Network-Assisted Molecular Dynamics
Lesheng Li, Marcos F. Calegari Andrade, Roberto Car, Annabella Selloni, Emily A. Carter
J. Phys. Chem. C, 2023, 127, 9750–9758.
DOI: 10.1021/acs.jpcc.2c08581
An accurate interatomic potential for the TiAlNb ternary alloy developed by deep neural network learning method
Jiajun Lu, Jinkai Wang, Kaiwei Wan, Ying Chen, Hao Wang, Xinghua Shi
J. Chem. Phys., 2023, 158.
DOI: 10.1063/5.0147720
In Silico Demonstration of Fast Anhydrous Proton Conduction on Graphanol
Siddarth K Achar, Leonardo Bernasconi, Ruby I DeMaio, Katlyn R Howard, J Karl Johnson
ACS Appl. Mater. Interfaces, 2023, 15, 25873–25883.
DOI: 10.1021/acsami.3c04022
Machine learning insight into h-BN growth on Pt(111) from atomic states
Kangmo Yeo, Sukmin Jeong
Applied Surface Science, 2023, 621, 156893.
DOI: 10.1016/j.apsusc.2023.156893
Molecular dynamics simulations of CaCl2-NaCl molten salt based on the machine learning potentials
Yun Xie, Min Bu, Guiming Zou, Ye Zhang, Guimin Lu
Solar Energy Materials and Solar Cells, 2023, 254, 112275.
DOI: 10.1016/j.solmat.2023.112275
A Heterogeneous Parallel Non-von Neumann Architecture System for Accurate and Efficient Machine Learning Molecular Dynamics
Zhuoying Zhao, Ziling Tan, Pinghui Mo, Xiaonan Wang, Dan Zhao, Xin Zhang, Ming Tao, Jie Liu
IEEE Trans. Circuits Syst. I, 2023, 70, 2439–2449.
DOI: 10.1109/TCSI.2023.3255199
Noble gas (He, Ne, and Ar) solubilities in high-pressure silicate melts calculated based on deep-potential modeling
Kai Wang, Xiancai Lu, Xiandong Liu, Kun Yin
Geochimica et Cosmochimica Acta, 2023, 350, 57–68.
DOI: 10.1016/j.gca.2023.03.032
Reversible densification and cooperative atomic movement induced \textquotedblleftcompaction\textquotedblright in vitreous silica: a new sight from deep neural network interatomic potentials
Yongnian Qi, Xiaoguang Guo, Hao Wang, Shuohua Zhang, Ming Li, Ping Zhou, Dongming Guo
J Mater Sci, 2023, 58, 9515–9532.
DOI: 10.1007/s10853-023-08599-w
Molecular dynamics simulation of Fe-Si alloys using a neural network machine learning potential
Huaijun Sun, Chao Zhang, Ling Tang, Renhai Wang, Weiyi Xia, Cai-Zhuang Wang
Phys. Rev. B, 2023, 107, 224301.
DOI: 10.1103/PhysRevB.107.224301
Evaluation of Machine Learning Interatomic Potentials for Gold Nanoparticles\textemdashTransferability towards Bulk
Marco Fronzi, Roger D Amos, Rika Kobayashi
Nanomaterials (Basel)., 2023, 13, 1832.
DOI: 10.3390/nano13121832
A genome dependence of metastable phase selection on atomic structure for undercooled liquid Nb90Si10 hypoeutectic alloy
Q. Wang, C. H. Zheng, M. X. Li, L. Hu, H. P. Wang, B. Wei
2023, 122.
DOI: 10.1063/5.0152293
TimeSOAP: Tracking high-dimensional fluctuations in complex molecular systems via time variations of SOAP spectra
Cristina Caruso, Annalisa Cardellini, Martina Crippa, Daniele Rapetti, Giovanni M Pavan
J. Chem. Phys., 2023, 158.
DOI: 10.1063/5.0147025
Deciphering the Anomalous Acidic Tendency of Terminal Water at Rutile(110)-Water Interfaces
Yong-Bin Zhuang, Jun Cheng
J. Phys. Chem. C, 2023, 127, 10532–10540.
DOI: 10.1021/acs.jpcc.3c01870
Temperature-dependent microwave dielectric permittivity of gallium oxide: A deep potential molecular dynamics study
Zhiqiang Li, Xinlei Duan, Linhua Liu, Jia-Yue Yang
2023, 133.
DOI: 10.1063/5.0149447
Investigating the Hydroxyl Reorientation in Hydroxyapatite Using Machine Learning Potentials
Jing Wang, Xin Wang, Hua Zhu, Dingguo Xu
J. Phys. Chem. C, 2023, 127, 11369–11377.
DOI: 10.1021/acs.jpcc.3c02426
Probing Confinement Effects on the Infrared Spectra of Water with Deep Potential Molecular Dynamics Simulations
Marcos F Calegari Andrade, Tuan Anh Pham
J. Phys. Chem. Lett., 2023, 14, 5560–5566.
DOI: 10.1021/acs.jpclett.3c01054
A Deep Neural Network Potential to Study the Thermal Conductivity of MnBi2Te4 and Bi2Te3/MnBi2Te4 Superlattice
Ruijin Qu, Yawei Lv, Zhihong Lu
J. Electron. Mater., 2023, 52, 4475–4483.
DOI: 10.1007/s11664-023-10403-z
Molecular insight into the GaP(110)-water interface using machine learning accelerated molecular dynamics
Xue-Ting Fan, Xiao-Jian Wen, Yong-Bin Zhuang, Jun Cheng
Journal of Energy Chemistry, 2023, 82, 239–247.
DOI: 10.1016/j.jechem.2023.03.013
Modelling of dislocations, twins and crack-tips in HCP and BCC Ti
Tongqi Wen, Anwen Liu, Rui Wang, Linfeng Zhang, Jian Han, Han Wang, David J. Srolovitz, Zhaoxuan Wu
International Journal of Plasticity, 2023, 166, 103644.
DOI: 10.1016/j.ijplas.2023.103644
Halide Vacancies Create No Charge Traps on Lead Halide Perovskite Surfaces but Can Generate Deep Traps in the Bulk
Jingyi Ran, Bipeng Wang, Yifan Wu, Dongyu Liu, Carlos Mora Perez, Andrey S Vasenko, Oleg V Prezhdo
J. Phys. Chem. Lett., 2023, 14, 6028–6036.
DOI: 10.1021/acs.jpclett.3c01231
High-Throughput Condensed-Phase Hybrid Density Functional Theory for Large-Scale Finite-Gap Systems: The SeA Approach
Hsin-Yu Ko, Marcos F Calegari Andrade, Zachary M Sparrow, Ju-An Zhang, Robert A DiStasio Jr
J. Chem. Theory Comput., 2023, 19, 4182–4201.
DOI: 10.1021/acs.jctc.2c00827
Microscopic Mechanism of Proton Transfer in Pure Water under Ambient Conditions
Jun Huo, Jianghao Chen, Pei Liu, Benkun Hong, Jian Zhang, Hao Dong, Shuhua Li
J. Chem. Theory Comput., 2023, 19, 4243–4254.
DOI: 10.1021/acs.jctc.3c00244
Revealing Carbon Vacancy Distribution on $\alpha$-MoC1-x Surfaces by Machine-Learning Force-Field-Aided Cluster Expansion Approach
Jun-Zhong Xie, Hong Jiang
J. Phys. Chem. C, 2023, 127, 13228–13237.
DOI: 10.1021/acs.jpcc.3c01941
Modeling the aqueous interface of amorphous TiO2 using deep potential molecular dynamics
Zhutian Ding, Annabella Selloni
J. Chem. Phys., 2023, 159.
DOI: 10.1063/5.0157188
Transferability evaluation of the deep potential model for simulating water-graphene confined system
Dongfei Liu, Jianzhong Wu, Diannan Lu
J. Chem. Phys., 2023, 159.
DOI: 10.1063/5.0153196
Structural transformations in single-crystalline AgPd nanoalloys from multiscale deep potential molecular dynamics
Longfei Guo, Tao Jin, Shuang Shan, Quan Tang, Zhen Li, Chongyang Wang, Junpeng Wang, Bowei Pan, Qiao Wang, Fuyi Chen
J. Chem. Phys., 2023, 159.
DOI: 10.1063/5.0158918
Detecting dynamic domains and local fluctuations in complex molecular systems via timelapse neighbors shuffling
Martina Crippa, Annalisa Cardellini, Cristina Caruso, Giovanni M Pavan
Proc. Natl. Acad. Sci. U. S. A., 2023, 120, e2300565120.
DOI: 10.1073/pnas.2300565120
Anisotropic Collective Variables with Machine Learning Potential for Ab Initio Crystallization of Complex Ceramics
Yuanpeng Deng, Shubin Fu, Jingran Guo, Xiang Xu, Hui Li
ACS Nano, 2023, 17, 14099–14113.
DOI: 10.1021/acsnano.3c04602
Fluorine spillover for ceria- vs silica-supported palladium nanoparticles: A MD study using machine learning potentials
Da-Jiang Liu, James W Evans
J. Chem. Phys., 2023, 159.
DOI: 10.1063/5.0147132
Rapid acquisition of liquid thermophysical properties from pure metals to quaternary alloys by proposing a machine learning strategy
R. L. Xiao, K. L. Liu, Y. Ruan, B. Wei
2023, 123.
DOI: 10.1063/5.0160046
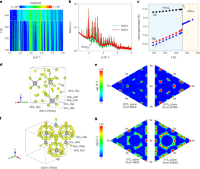
Extreme phonon anharmonicity underpins superionic diffusion and ultralow thermal conductivity in argyrodite Ag8SnSe6
Qingyong Ren, Mayanak K Gupta, Min Jin, Jingxuan Ding, Jiangtao Wu, Zhiwei Chen, Siqi Lin, Oscar Fabelo, Jose Alberto Rodr'\iguez-Velamaz'an, Maiko Kofu, Kenji Nakajima, Marcell Wolf, Fengfeng Zhu, Jianli Wang, Zhenxiang Cheng, Guohua Wang, Xin Tong, Yanzhong Pei, Olivier Delaire, Jie Ma
Nat. Mater., 2023, 22, 999–1006.
DOI: 10.1038/s41563-023-01560-x
Highly transferable atomistic machine-learning potentials from curated and compact datasets across the periodic table
Christopher M. Andolina, Wissam A. Saidi
Digital Discovery, 2023, 2, 1070–1077.
DOI: 10.1039/d3dd00046j
Unraveling the Oxidation Behaviors of MXenes in Aqueous Systems by Active-Learning-Potential Molecular-Dynamics Simulation
Pengfei Hou, Yumiao Tian, Yu Xie, Fei Du, Gang Chen, Aleksandra Vojvodic, Jianzhong Wu, Xing Meng
Angew. Chem. Int. Ed. Engl., 2023, 62, e202304205.
DOI: 10.1002/anie.202304205
DeePMD-kit v2: A software package for deep potential models
Jinzhe Zeng, Duo Zhang, Denghui Lu, Pinghui Mo, Zeyu Li, Yixiao Chen, Mari'an Rynik, Li'ang Huang, Ziyao Li, Shaochen Shi, Yingze Wang, Haotian Ye, Ping Tuo, Jiabin Yang, Ye Ding, Yifan Li, Davide Tisi, Qiyu Zeng, Han Bao, Yu Xia, Jiameng Huang, Koki Muraoka, Yibo Wang, Junhan Chang, Fengbo Yuan, Sigbj\orn L\oland Bore, Chun Cai, Yinnian Lin, Bo Wang, Jiayan Xu, Jia-Xin Zhu, Chenxing Luo, Yuzhi Zhang, Rhys E A Goodall, Wenshuo Liang, Anurag Kumar Singh, Sikai Yao, Jingchao Zhang, Renata Wentzcovitch, Jiequn Han, Jie Liu, Weile Jia, Darrin M York, Weinan E, Roberto Car, Linfeng Zhang, Han Wang
J. Chem. Phys., 2023, 159.
DOI: 10.1063/5.0155600
Melting curves of ice polymorphs in the vicinity of the liquid-liquid critical point
Pablo M Piaggi, Thomas E Gartner, Roberto Car, Pablo G Debenedetti
J. Chem. Phys., 2023, 159.
DOI: 10.1063/5.0159288
Spontaneous Hybrid Nano-Domain Behavior of the Organic-Inorganic Hybrid Perovskites
Ping Tuo, Lei Li, Xiaoxu Wang, Jianhui Chen, Zhicheng Zhong, Bo Xu, Fu-Zhi Dai
Adv Funct Materials, 2023, 33.
DOI: 10.1002/adfm.202301663
Thermal Conductivity of Water at Extreme Conditions
Cunzhi Zhang, Marcello Puligheddu, Linfeng Zhang, Roberto Car, Giulia Galli
J. Phys. Chem. B, 2023, 127, 7011–7.
DOI: 10.1021/acs.jpcb.3c02972
Local structure, thermodynamics, and melting of boron phosphide at high pressures by deep learning-driven ab~initio simulations
N M Chtchelkatchev, R E Ryltsev, M V Magnitskaya, S M Gorbunov, K A Cherednichenko, V L Solozhenko, V V Brazhkin
J. Chem. Phys., 2023, 159.
DOI: 10.1063/5.0165948
Exploring Configurations of Nanocrystal Ligands Using Machine-Learned Force Fields
Jakub K Sowa, Sean T Roberts, Peter J Rossky
J. Phys. Chem. Lett., 2023, 14, 7215–7222.
DOI: 10.1021/acs.jpclett.3c01618
Deep Potential Molecular Dynamics Study of Chapman-Jouguet Detonation Events of Energetic Materials
Jidong Zhang, Wei Guo, Yugui Yao
J. Phys. Chem. Lett., 2023, 14, 7141–7148.
DOI: 10.1021/acs.jpclett.3c01392
Why Dissolving Salt in Water Decreases Its Dielectric Permittivity
Chunyi Zhang, Shuwen Yue, Athanassios Z Panagiotopoulos, Michael L Klein, Xifan Wu
Phys. Rev. Lett., 2023, 131, 76801.
DOI: 10.1103/PhysRevLett.131.076801
Characterization of the Hydrogen-Bond Network in High-Pressure Water by Deep Potential Molecular Dynamics
Renxi Liu, Mohan Chen
J. Chem. Theory Comput., 2023, 19, 5602–5608.
DOI: 10.1021/acs.jctc.3c00445
Equation of state predictions for ScF3 and CaZrF6 with neural network- driven molecular dynamics
John P Stoppelman, Angus P Wilkinson, Jesse G McDaniel
J. Chem. Phys., 2023, 159.
DOI: 10.1063/5.0157615
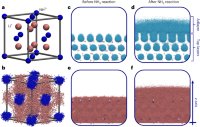
Reactant-induced dynamics of lithium imide surfaces during the ammonia decomposition process
Manyi Yang, Umberto Raucci, Michele Parrinello
Nat Catal, 2023, 6, 829–836.
DOI: 10.1038/s41929-023-01006-2
Deep neural network potential for simulating hydrogen blistering in tungsten
Xiao-Yang Wang, Yi-Nan Wang, Ke Xu, Fu-Zhi Dai, Hai-Feng Liu, Guang-Hong Lu, Han Wang
Phys. Rev. Materials, 2023, 7, 93601.
DOI: 10.1103/PhysRevMaterials.7.093601
ChecMatE: A workflow package to automatically generate machine learning potentials and phase diagrams for semiconductor alloys
Yu-Xin Guo, Yong-Bin Zhuang, Jueli Shi, Jun Cheng
J. Chem. Phys., 2023, 159.
DOI: 10.1063/5.0166858
Unraveling the Atomic-scale Mechanism of Phase Transformations and Structural Evolutions during (de)Lithiation in Si Anodes
Fangjia Fu, Xiaoxu Wang, Linfeng Zhang, Yifang Yang, Jianhui Chen, Bo Xu, Chuying Ouyang, Shenzhen Xu, Fu-Zhi Dai, Weinan E
Adv Funct Materials, 2023, 33.
DOI: 10.1002/adfm.202303936
High-Accuracy Machine-Learned Interatomic Potentials for the Phase Change Material Ge3Sb6Te5
Wei Yu, Zhaofu Zhang, Xuhao Wan, Jinhao Su, Qingzhong Gui, Hailing Guo, Hong-xia Zhong, John Robertson, Yuzheng Guo
Chem. Mater., 2023, 35, 6651–6658.
DOI: 10.1021/acs.chemmater.3c00524
Distinct anharmonic characteristics of phonon-driven lattice thermal conductivity and thermal expansion in bulk MoSe2 and WSe2
Mayanak K. Gupta, Sajan Kumar, Ranjan Mittal, Sanjay K. Mishra, Stephane Rols, Olivier Delaire, Arumugum Thamizhavel, P. U. Sastry, Samrath L. Chaplot
J. Mater. Chem. A, 2023, 11, 21864–21873.
DOI: 10.1039/d3ta03830k
Deciphering the Atomistic Mechanism of Si(111)-7 \texttimes 7 Surface Reconstruction Using a Machine-Learning Force Field
Yidi Shen, Sergey I Morozov, Kun Luo, Qi An, William A Goddard Iii
J. Am. Chem. Soc., 2023, 145, 20511–20520.
DOI: 10.1021/jacs.3c06540
Large scale and quantum accurate molecular dynamics simulation: Liquid iron under extreme condition
Qi-Yu Zeng, Bo Chen, Dong-Dong Kang, Jia-Yu Dai
Acta Phys. Sin., 2023, 72, 187102.
DOI: 10.7498/aps.72.20231258
Role of hydrogen-doping for compensating oxygen-defect in non- stoichiometric amorphous In2O3$-$x: Modeling with a machine-learning potential
Shingo Urata, Nobuhiro Nakamura, Junghwan Kim, Hideo Hosono
2023, 134.
DOI: 10.1063/5.0149199
Advancing Accurate and Efficient Surface Behavior Modeling of Al Clusters with Machine Learning Potential
Chongteng Wu, Tong Liu, Xiayu Ran, Yuefeng Su, Yun Lu, Ning Li, Lai Chen, Zhenwei Wu, Feng Wu, Duanyun Cao
J. Phys. Chem. C, 2023, 127, 19115–19126.
DOI: 10.1021/acs.jpcc.3c03229
Atomistic modeling of lithium materials from deep learning potential with ab initio accuracy
Haidi Wang, Tao Li, Yufan Yao, Xiaofeng Liu, Weiduo Zhu, Zhao Chen, Zhongjun Li, Wei Hu
2023, 36, 573–581.
DOI: 10.1063/1674-0068/cjcp2211173
Collective motion in hcp-Fe at Earth\textquoterights inner core conditions
Youjun Zhang, Yong Wang, Yuqian Huang, Junjie Wang, Zhixin Liang, Long Hao, Zhipeng Gao, Jun Li, Qiang Wu, Hong Zhang, Yun Liu, Jian Sun, Jung-Fu Lin
Proc. Natl. Acad. Sci. U. S. A., 2023, 120, e2309952120.
DOI: 10.1073/pnas.2309952120
Machine-Learning Accelerated First-Principles Accurate Modeling of the Solid-Liquid Phase Transition in MgO under Mantle Conditions
Pandu Wisesa, Christopher M Andolina, Wissam A Saidi
J. Phys. Chem. Lett., 2023, 14, 8741–8748.
DOI: 10.1021/acs.jpclett.3c02424
Thermal transport across TiO2-H2O interface involving water dissociation: Ab initio-assisted deep potential molecular dynamics
Zhiqiang Li, Jian Wang, Chao Yang, Linhua Liu, Jia-Yue Yang
J. Chem. Phys., 2023, 159.
DOI: 10.1063/5.0167238
Deep-learning-assisted theoretical insights into the compatibility of environment friendly insulation medium with metal surface of power equipment
Xuhao Wan, Zhaofu Zhang, Anyang Wang, Jinhao Su, Wenjun Zhou, John Robertson, Yuan Peng, Yu Zheng, Yuzheng Guo
J. Colloid Interface Sci., 2023, 648, 317–326.
DOI: 10.1016/j.jcis.2023.05.188
Computational study of Na diffusion and conduction in P2- and O3-Na2x[NixTi1-x]O2 materials with machine-learning interatomic potentials
Yining He, Qian Chen, Wei Lai
Solid State Ionics, 2023, 399, 116298.
DOI: 10.1016/j.ssi.2023.116298
Self-powered H2 generation implemented by hydrazine oxidation assisting hybrid electrochemical cell
Xi Liu, Wei Sun, Xiang Hu, Junxiang Chen, Zhenhai Wen
Chemical Engineering Journal, 2023, 474, 145355.
DOI: 10.1016/j.cej.2023.145355
Understanding the sluggish and highly variable transport kinetics of lithium ions in LiFePO4
Youcheng Hu, Xiaoxiao Wang, Peng Li, Junxiang Chen, Shengli Chen
Sci. China Chem., 2023, 66, 3297–3306.
DOI: 10.1007/s11426-023-1662-9
Thermal conductivity of Fe-bearing bridgmanite and post-perovskite: Implications for the heat flux from the core
Dong Wang, Zhongqing Wu, Xin Deng
Earth and Planetary Science Letters, 2023, 621, 118368.
DOI: 10.1016/j.epsl.2023.118368
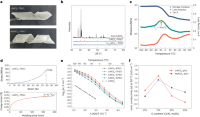
Inorganic glass electrolytes with polymer-like viscoelasticity
Tao Dai, Siyuan Wu, Yaxiang Lu, Yang Yang, Yuan Liu, Chao Chang, Xiaohui Rong, Ruijuan Xiao, Junmei Zhao, Yanhui Liu, Weihua Wang, Liquan Chen, Yong-Sheng Hu
Nat Energy, 2023, 8, 1221–1228.
DOI: 10.1038/s41560-023-01356-y
Machine learning potential for Ab Initio phase transitions of zirconia
Yuanpeng Deng, Chong Wang, Xiang Xu, Hui Li
Theoretical and Applied Mechanics Letters, 2023, 13, 100481.
DOI: 10.1016/j.taml.2023.100481
Modelling electrified microporous carbon/electrolyte electrochemical interface and unraveling charge storage mechanism by machine learning accelerated molecular dynamics
Yifeng Zhang, Hui Huang, Jie Tian, Chengwei Li, Yuchen Jiang, Zeng Fan, Lujun Pan
Energy Storage Materials, 2023, 63, 103069.
DOI: 10.1016/j.ensm.2023.103069
From ab~initio to continuum: Linking multiple scales using deep-learned forces
Haiyi Wu, Chenxing Liang, Jinu Jeong, N R Aluru
J. Chem. Phys., 2023, 159.
DOI: 10.1063/5.0166927
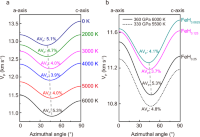
Superionic effect and anisotropic texture in Earth\textquoterights inner core driven by geomagnetic field
Shichuan Sun, Yu He, Junyi Yang, Yufeng Lin, Jinfeng Li, Duck Young Kim, Heping Li, Ho-Kwang Mao
Nat. Commun., 2023, 14, 1656.
DOI: 10.1038/s41467-023-37376-1
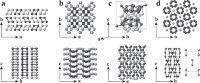
Data-driven prediction of complex crystal structures of dense lithium
Xiaoyang Wang, Zhenyu Wang, Pengyue Gao, Chengqian Zhang, Jian Lv, Han Wang, Haifeng Liu, Yanchao Wang, Yanming Ma
Nat. Commun., 2023, 14, 2924.
DOI: 10.1038/s41467-023-38650-y
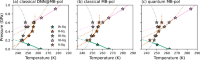
Realistic phase diagram of water from \textquotedblleftfirst principles\textquotedblright data-driven quantum simulations
Sigbj\orn L\oland Bore, Francesco Paesani
Nat. Commun., 2023, 14, 3349.
DOI: 10.1038/s41467-023-38855-1
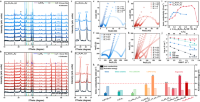
Realizing long-cycling all-solid-state Li-In||TiS2 batteries using Li6+xMxAs1-xS5I (M=Si, Sn) sulfide solid electrolytes
Pushun Lu, Yu Xia, Guochen Sun, Dengxu Wu, Siyuan Wu, Wenlin Yan, Xiang Zhu, Jiaze Lu, Quanhai Niu, Shaochen Shi, Zhengju Sha, Liquan Chen, Hong Li, Fan Wu
Nat. Commun., 2023, 14, 4077.
DOI: 10.1038/s41467-023-39686-w
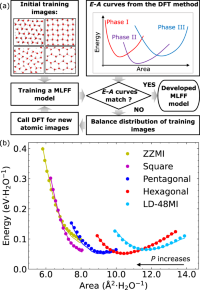
Temperature-pressure phase diagram of confined monolayer water/ice at first-principles accuracy with a machine-learning force field
Bo Lin, Jian Jiang, Xiao Cheng Zeng, Lei Li
Nat. Commun., 2023, 14, 4110.
DOI: 10.1038/s41467-023-39829-z
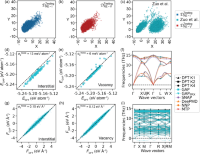
Discrepancies and error evaluation metrics for machine learning interatomic potentials
Yunsheng Liu, Xingfeng He, Yifei Mo
npj Comput Mater, 2023, 9, 174.
DOI: 10.1038/s41524-023-01123-3
Dislocation-mediated migration of the $\alpha$/$\beta$ interfaces in titanium
Jin-Yu Zhang, Zhi-Peng Sun, Dong Qiu, Fu-Zhi Dai, Yang- Sheng Zhang, Dongsheng Xu, Wen-Zheng Zhang
Acta Materialia, 2023, 261, 119364.
DOI: 10.1016/j.actamat.2023.119364
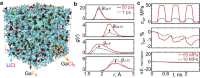
What dictates soft clay-like lithium superionic conductor formation from rigid salts mixture
Sunny Gupta, Xiaochen Yang, Gerbrand Ceder
Nat. Commun., 2023, 14, 6884.
DOI: 10.1038/s41467-023-42538-2
Interfacial heat and mass transfer at silica/binary molten salt interface from deep potential molecular dynamics
Fei Liang, Jing Ding, Xiaolan Wei, Gechuanqi Pan, Shule Liu
International Journal of Heat and Mass Transfer, 2023, 217, 124705.
DOI: 10.1016/j.ijheatmasstransfer.2023.124705
Reversible and irreversible photon-absorption in amorphous SiO2 revealed by deep potential
Yongnian Qi, Xiaoguang Guo, Ming Li, Chongkun Wang, Qing Mu, Ping Zhou
Journal of Non-Crystalline Solids, 2023, 622, 122682.
DOI: 10.1016/j.jnoncrysol.2023.122682
Thermal conductivity of van der Waals heterostructure of 2D GeS and SnS based on machine learning interatomic potential
Wentao Li, Chenxiu Yang
J. Phys. Condens. Matter, 2023, 35, 505001.
DOI: 10.1088/1361-648X/acf6ea
Development of Deep Potentials of Molten MgCl2-NaCl and MgCl2-KCl Salts Driven by Machine Learning
Tingrui Xu, Xuejiao Li, Yang Wang, Zhongfeng Tang
ACS Appl. Mater. Interfaces, 2023.
DOI: 10.1021/acsami.2c19272
Medium-entropy ceramic aerogels for robust thermal sealing
Shubin Fu, Dizhou Liu, Yuanpeng Deng, Menglin Li, Han Zhao, Jingran Guo, Jian Zhou, Pengyu Zhang, Chong Wang, Hongxuan Yu, Shixuan Dang, Jianing Zhang, Menglong Hao, Hui Li, Xiang Xu
J. Mater. Chem. A, 2023, 11, 742–752.
DOI: 10.1039/d2ta08264k
Grain boundaries induce significant decrease in lattice thermal conductivity of CdTe
Xiaona Huang, Kun Luo, Yidi Shen, Yanan Yue, Qi An
Energy and AI, 2023, 11, 100210.
DOI: 10.1016/j.egyai.2022.100210
Revisiting the structure, interaction, and dynamical property of ionic liquid from the deep learning force field
Yulong Ling, Kun Li, Mi Wang, Junfeng Lu, Chenlu Wang, Yanlei Wang, Hongyan He
Journal of Power Sources, 2023, 555, 232350.
DOI: 10.1016/j.jpowsour.2022.232350
Quasiplastic deformation in shocked nanocrystalline boron carbide: Grain boundary sliding and local amorphization
Jun Li, Qi An
Journal of the European Ceramic Society, 2023, 43, 208–216.
DOI: 10.1016/j.jeurceramsoc.2022.10.014
Accurate Fe-He machine learning potential for studying He effects in BCC-Fe
Krishna Chaitanya Pitike, Wahyu Setyawan
Journal of Nuclear Materials, 2023, 574, 154183.
DOI: 10.1016/j.jnucmat.2022.154183
Solvation structures of calcium and magnesium ions in water with the presence of hydroxide: a study by deep potential molecular dynamics
Jianchuan Liu, Renxi Liu, Yu Cao, Mohan Chen
Phys. Chem. Chem. Phys., 2023.
DOI: 10.1039/d2cp04105g
Grain boundary sliding and distortion on a nanosecond timescale induce trap states in CsPbBr3: ab initio investigation with machine learning force field
Dongyu Liu, Yifan Wu, Andrey S Vasenko, Oleg V Prezhdo
Nanoscale, 2023, 15, 285–293.
DOI: 10.1039/d2nr05918e
Accurate interatomic potential for the nucleation in liquid Ti-Al binary alloy developed by deep neural network learning method
B. Zhai, H.P. Wang
Computational Materials Science, 2023, 216, 111843.
DOI: 10.1016/j.commatsci.2022.111843
2022
Convergence acceleration in machine learning potentials for atomistic simulations
Dylan Bayerl, Christopher M. Andolina, Shyam Dwaraknath, Wissam A. Saidi
Digital Discovery, 2022, 1, 61–69.
DOI: 10.1039/d1dd00005e
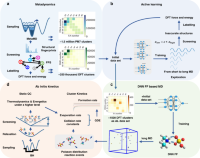
Towards fully ab initio simulation of atmospheric aerosol nucleation
Shuai Jiang, Yi-Rong Liu, Teng Huang, Ya-Juan Feng, Chun- Yu Wang, Zhong-Quan Wang, Bin-Jing Ge, Quan-Sheng Liu, Wei-Ran Guang, Wei Huang
Nat. Commun., 2022, 13, 6067.
DOI: 10.1038/s41467-022-33783-y
Thermoelectric Figure of Merit of a Superatomic Crystal ReSeI2 Monolayer
Tingwei Li, Peng-Hu Du, Ling Bai, Qiang Sun, Puru Jena
Phys. Rev. Applied, 2022, 18, 64067.
DOI: 10.1103/PhysRevApplied.18.064067
Deep learning molecular dynamics simulation on microwave high- temperature dielectric function of silicon nitride
Zhi-Qiang Li, Xiao-Yu Tan, Xin-Lei Duan, Jing-Yi Zhang, Jia-Yue Yang
Acta Phys. Sin., 2022, 71, 247803.
DOI: 10.7498/aps.71.20221002
Transferable Deep Learning Potential Reveals Intermediate-Range Ordering Effects in LiF-NaF-ZrF4 Molten Salt
Rajni Chahal, Santanu Roy, Martin Brehm, Shubhojit Banerjee, Vyacheslav Bryantsev, Stephen T Lam
JACS Au, 2022, 2, 2693–2702.
DOI: 10.1021/jacsau.2c00526
Elasticity and Viscosity of hcp Iron at Earth's Inner Core Conditions From Machine Learning-Based Large-Scale Atomistic Simulations
Zhi Li, Sandro Scandolo
Geophysical Research Letters, 2022, 49.
DOI: 10.1029/2022GL101161
Modeling Chemical Reactions in Alkali Carbonate-Hydroxide Electrolytes with Deep Learning Potentials
Anirban Mondal, Dina Kussainova, Shuwen Yue, Athanassios Z Panagiotopoulos
J. Chem. Theory Comput., 2022.
DOI: 10.1021/acs.jctc.2c00816
Spatial arrangement of dynamic surface species from solid-state NMR and machine learning-accelerated MD simulations
Takeshi Kobayashi, Da-Jiang Liu, Fr'ed'eric A Perras
Chem. Commun. (Camb)., 2022, 58, 13939–13942.
DOI: 10.1039/d2cc05861h
Fluctuations at Metal Halide Perovskite Grain Boundaries Create Transient Trap States: Machine Learning Assisted Ab Initio Analysis
Yifan Wu, Dongyu Liu, Weibin Chu, Bipeng Wang, Andrey S Vasenko, Oleg V Prezhdo
ACS Appl. Mater. Interfaces, 2022, 14, 55753–55761.
DOI: 10.1021/acsami.2c16203
Modeling Short-Range and Three-Membered Ring Structures in Lithium Borosilicate Glasses Using a Machine-Learning Potential
Shingo Urata
J. Phys. Chem. C, 2022, 126, 21507–21517.
DOI: 10.1021/acs.jpcc.2c07597
Lattice Thermal Conductivity of MgSiO3 Perovskite and Post- Perovskite under Lower Mantle Conditions Calculated by Deep Potential Molecular Dynamics
Fenghu Yang, Qiyu Zeng, Bo Chen, Dongdong Kang, Shen Zhang, Jianhua Wu, Xiaoxiang Yu, Jiayu Dai
Chinese Phys. Lett., 2022, 39, 116301.
DOI: 10.1088/0256-307X/39/11/116301
Origin of the herringbone reconstruction of Au(111) surface at the atomic scale
Pai Li, Feng Ding
Sci. Adv., 2022, 8, eabq2900.
DOI: 10.1126/sciadv.abq2900
Resolving the odd-even oscillation of water dissociation at rutile TiO2(110)-water interface by machine learning accelerated molecular dynamics
Yong-Bin Zhuang, Rui-Hao Bi, Jun Cheng
J. Chem. Phys., 2022, 157, 164701.
DOI: 10.1063/5.0126333
Evaluation of Machine Learning Interatomic Potentials for the Properties of Gold Nanoparticles
Marco Fronzi, Roger D Amos, Rika Kobayashi, Naoki Matsumura, Kenta Watanabe, Rafael K Morizawa
Nanomaterials (Basel)., 2022, 12, 3891.
DOI: 10.3390/nano12213891
Predicted superconductivity and superionic state in the electride Li5N under high pressure
Zhongyu Wan, Chao Zhang, Tianyi Yang, Wenjun Xu, Ruiqin Zhang
New J. Phys., 2022, 24, 113012.
DOI: 10.1088/1367-2630/ac9cff
Origin of negative thermal expansion and pressure-induced amorphization in zirconium tungstate from a machine-learning potential
Ri He, Hongyu Wu, Yi Lu, Zhicheng Zhong
Phys. Rev. B, 2022, 106, 174101.
DOI: 10.1103/PhysRevB.106.174101
Phonon Thermal Transport in Bi2Te3 from a Deep-Neural-Network Interatomic Potential
Robert D McMichael, Sean M Blakley
Phys. Rev. Appl., 2022, 18, 54022.
DOI: 10.1103/PhysRevApplied.18.054022
Piezo- and Pyroelectricity in Zirconia: A Study with Machine-Learned Force Fields
Robert D McMichael, Sean M Blakley
Phys. Rev. Appl., 2022, 18, 54066.
DOI: 10.1103/PhysRevApplied.18.054066
Classical and machine learning interatomic potentials for BCC vanadium
Rui Wang, Xiaoxiao Ma, Linfeng Zhang, Han Wang, David J. Srolovitz, Tongqi Wen, Zhaoxuan Wu
Phys. Rev. Materials, 2022, 6, 113603.
DOI: 10.1103/PhysRevMaterials.6.113603
Order of magnitude reduction in Joule heating of single molecular junctions between graphene electrodes
Gen Li, Bing-Zhong Hu, Wen-Hao Mao, Nuo Yang, Jing-Tao L"u
J. Chem. Phys., 2022, 157, 174303.
DOI: 10.1063/5.0118952
Plastic deformation of superionic water ices
Filipe Matusalem, J'essica Santos Rego, Maurice de Koning
Proc. Natl. Acad. Sci. U. S. A., 2022, 119, e2203397119.
DOI: 10.1073/pnas.2203397119
Metal Affinity of Support Dictates Sintering of Gold Catalysts
Jin-Cheng Liu, Langli Luo, Hai Xiao, Junfa Zhu, Yang He, Jun Li
J. Am. Chem. Soc., 2022, 144, 20601–20609.
DOI: 10.1021/jacs.2c06785
Multireference Generalization of the Weighted Thermodynamic Perturbation Method
Timothy J Giese, Jinzhe Zeng, Darrin M York
J. Phys. Chem. A, 2022, 126, 8519–8533.
DOI: 10.1021/acs.jpca.2c06201
Thermal Conductivity of Hydrous Wadsleyite Determined by Non-Equilibrium Molecular Dynamics Based on Machine Learning
Dong Wang, Zhongqing Wu, Xin Deng
Geophysical Research Letters, 2022, 49.
DOI: 10.1029/2022GL100337
DeePKS + ABACUS as a Bridge between Expensive Quantum Mechanical Models and Machine Learning Potentials
Wenfei Li, Qi Ou, Yixiao Chen, Yu Cao, Renxi Liu, Chunyi Zhang, Daye Zheng, Chun Cai, Xifan Wu, Han Wang, Mohan Chen, Linfeng Zhang
J. Phys. Chem. A, 2022, 126, 9154–9164.
DOI: 10.1021/acs.jpca.2c05000
Centroid Molecular Dynamics Can Be Greatly Accelerated through Neural Network Learned Centroid Forces Derived from Path Integral Molecular Dynamics
Timothy D Loose, Patrick G Sahrmann, Gregory A Voth
J. Chem. Theory Comput., 2022, 18, 5856–5863.
DOI: 10.1021/acs.jctc.2c00706
Moir'e Phonons in Magic-Angle Twisted Bilayer Graphene
Xiaoqian Liu, Ran Peng, Zhaoru Sun, Jianpeng Liu
Nano Lett., 2022, 22, 7791–7797.
DOI: 10.1021/acs.nanolett.2c02010
Modeling the Solvation and Acidity of Carboxylic Acids Using an Ab Initio Deep Neural Network Potential
Abhinav S Raman, Annabella Selloni
J. Phys. Chem. A, 2022, 126, 7283–7290.
DOI: 10.1021/acs.jpca.2c06252
Photoelectron spectra of water and simple aqueous solutions at extreme conditions
Zifan Ye, Cunzhi Zhang, Giulia Galli
Faraday Discuss., 2022, 236, 352–363.
DOI: 10.1039/d2fd00003b
Deep potential for a face-centered cubic Cu system at finite temperatures
Yunzhen Du, Zhaocang Meng, Qiang Yan, Canglong Wang, Yuan Tian, Wenshan Duan, Sheng Zhang, Ping Lin
Phys. Chem. Chem. Phys., 2022, 24, 18361–18369.
DOI: 10.1039/D2CP02758E
Thermal transport properties of monolayer GeS and SnS: A comparative study based on machine learning and SW interatomic potential models
Wentao Li, Chenxiu Yang
AIP Advances, 2022, 12, 85111.
DOI: 10.1063/5.0099448
Structural and electrocatalytic properties of copper clusters: A study via deep learning and first principles
Xiaoning Wang, Haidi Wang, Qiquan Luo, Jinlong Yang
J. Chem. Phys., 2022, 157, 74304.
DOI: 10.1063/5.0100505
A Deep Neural Network Interface Potential for Li-Cu Systems
Genming Lai, Junyu Jiao, Chi Fang, Ruiqi Zhang, Xianqi Xu, Liyuan Sheng, Yao Jiang, Chuying Ouyang, Jiaxin Zheng
Adv Materials Inter, 2022, 9, 2201346.
DOI: 10.1002/admi.202201346
Strategy to consider element distribution when constructing training datasets for developing machine learning potentials of alloys based on a Monte-Carlo-like method
Zhipeng Zhang, Liuqing Chen, Junyi Guo, Xianyin Duan, Bin Shan, Xianbao Duan
Phys. Rev. B, 2022, 106, 94107.
DOI: 10.1103/PhysRevB.106.094107
Combining Machine Learning Approaches and Accurate Ab Initio Enhanced Sampling Methods for Prebiotic Chemical Reactions in Solution
Timoth'ee Devergne, Th'eo Magrino, Fabio Pietrucci, A Marco Saitta
J. Chem. Theory Comput., 2022, 18, 5410–5421.
DOI: 10.1021/acs.jctc.2c00400
GPUMD: A package for constructing accurate machine-learned potentials and performing highly efficient atomistic simulations
Zheyong Fan, Yanzhou Wang, Penghua Ying, Keke Song, Junjie Wang, Yong Wang, Zezhu Zeng, Ke Xu, Eric Lindgren, J Magnus Rahm, Alexander J Gabourie, Jiahui Liu, Haikuan Dong, Jianyang Wu, Yue Chen, Zheng Zhong, Jian Sun, Paul Erhart, Yanjing Su, Tapio Ala-Nissila
J. Chem. Phys., 2022, 157, 114801.
DOI: 10.1063/5.0106617
Magnetocaloric effect in ScGdTbDyHo high-entropy alloy: Impact of synthesis route
S.A. Uporov, S. Kh Estemirova, E.V. Sterkhov, I.A. Balyakin, A.A. Rempel
Intermetallics, 2022, 151, 107678.
DOI: 10.1016/j.intermet.2022.107678
A tungsten deep neural-network potential for simulating mechanical property degradation under fusion service environment
Xiaoyang Wang, Yinan Wang, Linfeng Zhang, Fuzhi Dai, Han Wang
Nucl. Fusion, 2022, 62, 126013.
DOI: 10.1088/1741-4326/ac888b
Molecular dynamics simulations of LiCl ion pairs in high temperature aqueous solutions by deep learning potential
Wei Zhang, Li Zhou, Bin Yang, Tinggui Yan
Journal of Molecular Liquids, 2022, 367, 120500.
DOI: 10.1016/j.molliq.2022.120500
DP Compress: A Model Compression Scheme for Generating Efficient Deep Potential Models
Denghui Lu, Wanrun Jiang, Yixiao Chen, Linfeng Zhang, Weile Jia, Han Wang, Mohan Chen
J. Chem. Theory Comput., 2022, 18, 5555–5567.
DOI: 10.1021/acs.jctc.2c00102
Combining Machine Learning Approaches and Accurate Ab Initio Enhanced Sampling Methods for Prebiotic Chemical Reactions in Solution
Timoth'ee Devergne, Th'eo Magrino, Fabio Pietrucci, A Marco Saitta
J. Chem. Theory Comput., 2022.
DOI: 10.1021/acs.jctc.2c00400
A Deep Neural Network Interface Potential for Li-Cu Systems
Genming Lai, Junyu Jiao, Chi Fang, Ruiqi Zhang, Xianqi Xu, Liyuan Sheng, Yao Jiang, Chuying Ouyang, Jiaxin Zheng
Adv Materials Inter, 2022, 2201346.
DOI: 10.1002/admi.202201346
Nucleation of Water Clusters in Gas Phase: A Computational Study Based on Neural Network Potential and Enhanced Sampling\textreferencemark
Sen Xu, Liling Wu, Zhenyu Li
Acta Chimica Sinica, 2022, 80, 598.
DOI: 10.6023/A22010003
Toward High-level Machine Learning Potential for Water Based on Quantum Fragmentation and Neural Networks
Jinfeng Liu, Jinggang Lan, Xiao He
J. Phys. Chem. A, 2022, 126, 3926–3936.
DOI: 10.1021/acs.jpca.2c00601
A Deep Neural Network Potential for Water Confined in Graphene Nanocapillaries
Wen Zhao, Hu Qiu, Wanlin Guo
J. Phys. Chem. C, 2022, 126, 10546–10553.
DOI: 10.1021/acs.jpcc.2c02423
Soft-phonon anharmonicity, floppy modes, and Na diffusion in Na3FY (Y=S,Se,Te): Ab initio and machine-learned molecular dynamics simulations
Mayanak Kumar Gupta, Sajan Kumar, Ranjan Mittal, Samrath L. Chaplot
Phys. Rev. B, 2022, 106, 14311.
DOI: 10.1103/PhysRevB.106.014311
Combined QM/MM, Machine Learning Path Integral Approach to Compute Free Energy Profiles and Kinetic Isotope Effects in RNA Cleavage Reactions
Timothy J Giese, Jinzhe Zeng, Şölen Ekesan, Darrin M York
J. Chem. Theory Comput., 2022, 18, 4304–4317.
DOI: 10.1021/acs.jctc.2c00151
Automated workflow for computation of redox potentials, acidity constants, and solvation free energies accelerated by machine learning
Feng Wang, Jun Cheng
J. Chem. Phys., 2022, 157, 24103.
DOI: 10.1063/5.0098330
Homogeneous ice nucleation in an ab initio machine-learning model of water
Pablo M Piaggi, Jack Weis, Athanassios Z Panagiotopoulos, Pablo G Debenedetti, Roberto Car
Proc. Natl. Acad. Sci. U. S. A., 2022, 119, e2207294119.
DOI: 10.1073/pnas.2207294119
High accuracy neural network interatomic potential for NiTi shape memory alloy
Hao Tang, Yin Zhang, Qing-Jie Li, Haowei Xu, Yuchi Wang, Yunzhi Wang, Ju Li
Acta Materialia, 2022, 238, 118217.
DOI: 10.1016/j.actamat.2022.118217
Machine Learning Accelerates Molten Salt Simulations: Thermal Conductivity of MgCl 2 -NaCl Eutectic
Wenshuo Liang, Guimin Lu, Jianguo Yu
Advcd Theory and Sims, 2022, 2200206.
DOI: 10.1002/adts.202200206
Machine Learning Force Field Aided Cluster Expansion Approach to Configurationally Disordered Materials: Critical Assessment of Training Set Selection and Size Convergence
Jun-Zhong Xie, Xu-Yuan Zhou, Dong Luan, Hong Jiang
J. Chem. Theory Comput., 2022, 18, 3795–3804.
DOI: 10.1021/acs.jctc.2c00017
Combined Deep Learning and Classical Potential Approach for Modeling Diffusion in UiO-66
Siddarth K Achar, Jacob J Wardzala, Leonardo Bernasconi, Linfeng Zhang, J Karl Johnson
J. Chem. Theory Comput., 2022, 18, 3593–3606.
DOI: 10.1021/acs.jctc.2c00010
Deep neural network based quantum simulations and quasichemical theory for accurate modeling of molten salt thermodynamics
Yu Shi, Stephen T. Lam, Thomas~L. Beck
Chem. Sci., 2022.
DOI: 10.1039/D2SC02227C
Towards large-scale and spatiotemporally resolved diagnosis of electronic density of states by deep learning
Qiyu Zeng, Bo Chen, Xiaoxiang Yu, Shen Zhang, Dongdong Kang, Han Wang, Jiayu Dai
Phys. Rev. B, 2022, 105, 174109.
DOI: 10.1103/PhysRevB.105.174109
Exploring Complex Reaction Networks Using Neural Network-Based Molecular Dynamics Simulation
Qingzhao Chu, Kai H Luo, Dongping Chen
J. Phys. Chem. Lett., 2022, 13, 4052–4057.
DOI: 10.1021/acs.jpclett.2c00647
Reaction processes at step edges on S-decorated Cu(111) and Ag(111) surfaces: MD analysis utilizing machine learning derived potentials
Da-Jiang Liu, James W Evans
J. Chem. Phys., 2022, 156, 204106.
DOI: 10.1063/5.0089210
Deep machine learning potential for atomistic simulation of Fe-Si-O systems under Earth's outer core conditions
Chao Zhang, Ling Tang, Yang Sun, Kai-Ming Ho, Renata M. Wentzcovitch, Cai-Zhuang Wang
Phys. Rev. Materials, 2022, 6, 63802.
DOI: 10.1103/PhysRevMaterials.6.063802
Accelerated Deep Learning Dynamics for Atomic Layer Deposition of Al(Me)3 and Water on OH/Si(111)
Hiroya Nakata, Michael Filatov Gulak, Cheol Ho Choi
ACS Appl. Mater. Interfaces, 2022, 14, 26116–26127.
DOI: 10.1021/acsami.2c01768
Acids at the Edge: Why Nitric and Formic Acid Dissociations at Air-Water Interfaces Depend on Depth and on Interface Specific Area
Miguel de la Puente, Rolf David, Axel Gomez, Damien Laage
J. Am. Chem. Soc., 2022, 144, 10524–10529.
DOI: 10.1021/jacs.2c03099
Strongly Anharmonic Phonons and Their Role in Superionic Diffusion and Ultralow Thermal Conductivity of Cu 7 PSe 6
Mayanak K. Gupta, Jingxuan Ding, Dipanshu Bansal, Douglas L. Abernathy, Georg Ehlers, Naresh C. Osti, Wolfgang G. Zeier, Olivier Delaire
Advanced Energy Materials, 2022, 12, 2200596.
DOI: 10.1002/aenm.202200596
Atomistic Calculation of the Melting Point of the High-Entropy Cantor Alloy CoCrFeMnNi
I. A. Balyakin, A. A. Rempel
Dokl Phys Chem, 2022, 502, 11–17.
DOI: 10.1134/S0012501622010018
Deep potential development of transition-metal-rich carbides
Tyler McGilvry-James, Bikash Timalsina, Marium Mostafiz Mou, Ridwan Sakidja
MRS Advances, 2022, 7, 468–473.
DOI: 10.1557/s43580-022-00289-0
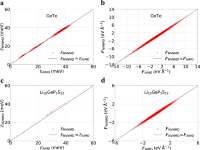
Accurate and efficient molecular dynamics based on machine learning and non von Neumann architecture
Pinghui Mo, Chang Li, Dan Zhao, Yujia Zhang, Mengchao Shi, Junhua Li, Jie Liu
npj Comput Mater, 2022, 8, 107.
DOI: 10.1038/s41524-022-00773-z
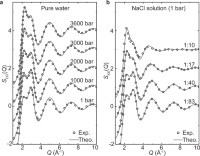
Dissolving salt is not equivalent to applying a pressure on water
Chunyi Zhang, Shuwen Yue, Athanassios Z Panagiotopoulos, Michael L Klein, Xifan Wu
Nat. Commun., 2022, 13, 822.
DOI: 10.1038/s41467-022-28538-8
Exploring the Effects of Ionic Defects on the Stability of CsPbI 3 with a Deep Learning Potential
Weijie Yang, Jiajia Li, Xuelu Chen, Yajun Feng, Chongchong Wu, Ian D Gates, Zhengyang Gao, Xunlei Ding, Jianxi Yao, Hao Li
Chemphyschem, 2022, 23, e202100841.
DOI: 10.1002/cphc.202100841
Structural phase transitions in $\mathrmSrTi\mathrmO_3$ from deep potential molecular dynamics
Ri He, Hongyu Wu, Linfeng Zhang, Xiaoxu Wang, Fangjia Fu, Shi Liu, Zhicheng Zhong
Phys. Rev. B, 2022, 105, 064104.
DOI: 10.1103/PhysRevB.105.064104

Efficient and accurate atomistic modeling of dopant migration using deep neural network
Xi Ding, Ming Tao, Junhua Li, Mingyuan Li, Mengchao Shi, Jiashu Chen, Zhen Tang, Francis Benistant, Jie Liu
Materials Science in Semiconductor Processing, 2022, 143, 106513.
DOI: 10.1016/j.mssp.2022.106513

Self-Healing Mechanism of Lithium in Lithium Metal
Junyu Jiao, Genming Lai, Liang Zhao, Jiaze Lu, Qidong Li, Xianqi Xu, Yao Jiang, Yan-Bing He, Chuying Ouyang, Feng Pan, Hong Li, Jiaxin Zheng
Adv. Sci. (Weinh)., 2022, 9, e2105574.
DOI: 10.1002/advs.202105574

A deep learning interatomic potential developed for atomistic simulation of carbon materials
Jinjin Wang, Hong Shen, Riyi Yang, Kun Xie, Chao Zhang, Liangyao Chen, Kai-Ming Ho, Cai-Zhuang Wang, Songyou Wang
Carbon, 2022, 186, 1–8.
DOI: 10.1016/j.carbon.2021.09.062

Using metadynamics to build neural network potentials for reactive events: the case of urea decomposition in water
Manyi Yang, Luigi Bonati, Daniela Polino, Michele Parrinello
Catalysis Today, 2022, 387, 143–149.
DOI: 10.1016/j.cattod.2021.03.018

Development of neural network potential for MD simulation and its application to TiN
Takeru Miyagawa, Kazuki Mori, Nobuhiko Kato, Akio Yonezu
Computational Materials Science, 2022, 206, 111303.
DOI: 10.1016/j.commatsci.2022.111303
Ab Initio Neural Network MD Simulation of Thermal Decomposition of High Energy Material CL-20/TNT
Liqun Cao, Jinzhe Zeng, Bo Wang, Tong Zhu, John Z.H. Zhang
Phys. Chem. Chem. Phys., 2022, 24, 11801–11811.
DOI: 10.1039/D2CP00710J
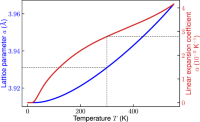
Temperature- and vacancy-concentration-dependence of heat transport in Li3ClO from multi-method numerical simulations
Paolo Pegolo, Stefano Baroni, Federico Grasselli
npj Comput Mater, 2022, 8, 24.
DOI: 10.1038/s41524-021-00693-4
Grain boundary segregation induced strong UHTCs at elevated temperatures: A universal mechanism from conventional UHTCs to high entropy UHTCs
Fu-Zhi Dai, Bo Wen, Yinjie Sun, Yixiao Ren, Huimin Xiang, Yanchun Zhou
Journal of Materials Science & Technology, 2022, 123, 26-33.
DOI: 10.1016/j.jmst.2021.12.074
The chemical origin of temperature-dependent lithium-ion concerted diffusion in sulfide solid electrolyte Li10GeP2S12
Zhong-Heng Fu, Xiang Chen, Nan Yao, Xin Shen, Xia-Xia Ma, Shuai Feng, Shuhao Wang, Rui Zhang, Linfeng Zhang, Qiang Zhang
Journal of Energy Chemistry, 2022, 70, 59–66.
DOI: 10.1016/j.jechem.2022.01.018
Study on the structural properties of refining slags by molecular dynamics with deep learning potential
Yuhan Sun, Min Tan, Tao Li, Junguo Li, Bo Shang
Journal of Molecular Liquids, 2022, 353, 118787.
DOI: 10.1016/j.molliq.2022.118787
Nanotwinning induced decreased lattice thermal conductivity of high temperature thermoelectric boron subphosphide (B12P2) from deep learning potential simulations
Xiaona Huang, Yidi Shen, Qi An
Energy and AI, 2022, 8, 100135.
DOI: 10.1016/j.egyai.2022.100135
A deep potential model with long-range electrostatic interactions
Linfeng Zhang, Han Wang, Maria Carolina Muniz, Athanassios Z Panagiotopoulos, Roberto Car, Weinan E
J. Chem. Phys., 2022, 156, 124107.
DOI: 10.1063/5.0083669
Four-Phonon Scattering Effect and Two-Channel Thermal Transport in Two-Dimensional Paraelectric SnSe
Jie Sun, Cunzhi Zhang, Zhonghua Yang, Yiheng Shen, Ming Hu, Qian Wang
ACS Appl. Mater. Interfaces, 2022, 14, 11493–11499.
DOI: 10.1021/acsami.1c24488
An inductive transfer learning force field (ITLFF) protocol builds protein force fields in seconds
Yanqiang Han, Zhilong Wang, An Chen, Imran Ali, Junfei Cai, Simin Ye, Jinjin Li
Brief. Bioinform., 2022, 23.
DOI: 10.1093/bib/bbab590
Sulfur-enhanced dynamics of coinage metal(111) surfaces: Step edges versus terraces as locations for metal-sulfur complex formation
Da-Jiang Liu, James W. Evans
Journal of Vacuum Science \& Technology A, 2022, 40 (2), 023205.
DOI: 10.1116/6.0001408
A generalizable machine learning potential of Ag-Au nanoalloys and its application to surface reconstruction, segregation and diffusion
YiNan Wang, LinFeng Zhang, Ben Xu, XiaoYang Wang, Han Wang
Modelling Simul. Mater. Sci. Eng., 2022, 30, 25003.
DOI: 10.1088/1361-651X/ac4002
Deep machine learning potentials for multicomponent metallic melts: Development, predictability and compositional transferability
R.E. Ryltsev, N.M. Chtchelkatchev
Journal of Molecular Liquids, 2022, 349, 118181.
DOI: 10.1016/j.molliq.2021.118181
Neural network potential for Zr-Rh system by machine learning
Kun Xie, Chong Qiao, Hong Shen, Riyi Yang, Ming Xu, Chao Zhang, Yuxiang Zheng, Rongjun Zhang, Liangyao Chen, Kai-Ming Ho, Cai-Zhuang Wang, Songyou Wang
J. Phys. Condens. Matter, 2022, 34, 75402.
DOI: 10.1088/1361-648X/ac37dc
Molecular dynamics simulation of molten strontium chloride based on deep potential
Di Guo, Jia Zhao, Wenshuo Liang, Guimin Lu
Journal of Molecular Liquids, 2022, 348, 118380.
DOI: 10.1016/j.molliq.2021.118380
Suppression of Rayleigh Scattering in Silica Glass by Codoping Boron and Fluorine: Molecular Dynamics Simulations with Force-Matching and Neural Network Potentials
Shingo Urata, Nobuhiro Nakamura, Tomofumi Tada, Aik Rui Tan, Rafael Gómez-Bombarelli, Hideo Hosono
J. Phys. Chem. C, 2022, 126 (4), 2264-2275.
DOI: 10.1021/acs.jpcc.1c10300
A deep learning potential applied in tobermorite phases and extended to calcium silicate hydrates
Yang Zhou, Haojie Zheng, Weihuan Li, Tao Ma, Changwen Miao
Cement and Concrete Research, 2022, 152, 106685.
DOI: 10.1016/j.cemconres.2021.106685
Deep learning potential for superionic phase of Ag2S
I.A. Balyakin, S.I. Sadovnikov
Computational Materials Science, 2022, 202, 110963.
DOI: 10.1016/j.commatsci.2021.110963
Neural network representation of electronic structure from ab initio molecular dynamics
Qiangqiang Gu, Linfeng Zhang, Ji Feng
Science Bulletin, 2022, 67, 29–37.
DOI: 10.1016/j.scib.2021.09.010
2021
Machine learning builds full-QM precision protein force fields in seconds
Yanqiang Han, Zhilong Wang, Zhiyun Wei, Jinyun Liu, Jinjin Li
Brief. Bioinform., 2021, 22.
DOI: 10.1093/bib/bbab158

Efficiently Trained Deep Learning Potential for Graphane
Siddarth K. Achar, Linfeng Zhang, J. Karl Johnson
J. Phys. Chem. C, 2021, 125, 14874–14882.
DOI: 10.1021/acs.jpcc.1c01411

2D Heterostructure of Amorphous CoFeB Coating Black Phosphorus Nanosheets with Optimal Oxygen Intermediate Absorption for Improved Electrocatalytic Water Oxidation
Huayu Chen, Junxiang Chen, Pei Ning, Xin Chen, Junhui Liang, Xin Yao, Da Chen, Laishun Qin, Yuexiang Huang, Zhenhai Wen
ACS Nano, 2021, 15, 12418–12428.
DOI: 10.1021/acsnano.1c04715

Deep potential generation scheme and simulation protocol for the Li10GeP2S12-type superionic conductors
Jianxing Huang, Linfeng Zhang, Han Wang, Jinbao Zhao, Jun Cheng, Weinan E
J. Chem. Phys., 2021, 154, 94703.
DOI: 10.1063/5.0041849

Machine-Learning-Assisted Free Energy Simulation of Solution-Phase and Enzyme Reactions
Xiaoliang Pan, Junjie Yang, Richard Van, Evgeny Epifanovsky, Junming Ho, Jing Huang, Jingzhi Pu, Ye Mei, Kwangho Nam, Yihan Shao
J. Chem. Theory Comput., 2021, 17, 5745–5758.
DOI: 10.1021/acs.jctc.1c00565
Accurate force field of two-dimensional ferroelectrics from deep learning
Jing Wu, Liyi Bai, Jiawei Huang, Liyang Ma, Jian Liu, Shi Liu
Phys. Rev. B, 2021, 104, 174107.
DOI: 10.1103/PhysRevB.104.174107

Exploring the Chemical Space of Linear Alkane Pyrolysis via Deep Potential GENerator
Jinzhe Zeng, Linfeng Zhang, Han Wang, Tong Zhu
Energy Fuels, 2021, 35, 762–769.
DOI: 10.1021/acs.energyfuels.0c03211

Development of Range-Corrected Deep Learning Potentials for Fast, Accurate Quantum Mechanical/Molecular Mechanical Simulations of Chemical Reactions in Solution
Jinzhe Zeng, Timothy J Giese, Şölen Ekesan, Darrin M York
J. Chem. Theory Comput., 2021, 17, 6993–7009.
DOI: 10.1021/acs.jctc.1c00201

Reactive uptake of N2O5 by atmospheric aerosol is dominated by interfacial processes
Mirza Galib, David T Limmer
Science, 2021, 371, 921–925.
DOI: 10.1126/science.abd7716

86 PFLOPS Deep Potential Molecular Dynamics simulation of 100 million atoms with ab initio accuracy
Denghui Lu, Han Wang, Mohan Chen, Lin Lin, Roberto Car, Weinan E, Weile Jia, Linfeng Zhang
Computer Physics Communications, 2021, 259, 107624.
DOI: 10.1016/j.cpc.2020.107624

Insights from Computational Studies on the Anisotropic Volume Change of LixNiO2 at High States of Charge (x < 0.25)
Juan C. Garcia, Joshua Gabriel, Noah H. Paulson, John Low, Marius Stan, Hakim Iddir
J. Phys. Chem. C, 2021, 125 (49), 27130-27139.
DOI: 10.1021/acs.jpcc.1c08022

Thermodynamic and Transport Properties of LiF and FLiBe Molten Salts with Deep Learning Potentials
Alejandro Rodriguez, Stephen Lam, Ming Hu
ACS Appl. Mater. Interfaces, 2021, 13, 55367–55379.
DOI: 10.1021/acsami.1c17942
Heat transport in liquid water from first-principles and deep neural network simulations
Davide Tisi, Linfeng Zhang, Riccardo Bertossa, Han Wang, Roberto Car, Stefano Baroni
Phys. Rev. B, 2021, 104, 224202.
DOI: 10.1103/PhysRevB.104.224202
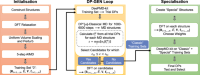
Specialising neural network potentials for accurate properties and application to the mechanical response of titanium
Tongqi Wen, Rui Wang, Lingyu Zhu, Linfeng Zhang, Han Wang, David J. Srolovitz, Zhaoxuan Wu
npj Comput Mater, 2021, 7, 206.
DOI: 10.1038/s41524-021-00661-y
Fast Na diffusion and anharmonic phonon dynamics in superionic Na3PS4
Mayanak K. Gupta, Jingxuan Ding, Naresh C. Osti, Douglas L. Abernathy, William Arnold, Hui Wang, Zachary Hood, Olivier Delaire
Energy Environ. Sci., 2021, 14, 6554-6563.
DOI: 10.1039/D1EE01509E
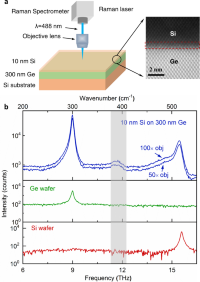
Experimental observation of localized interfacial phonon modes
Zhe Cheng, Ruiyang Li, Xingxu Yan, Glenn Jernigan, Jingjing Shi, Michael E Liao, Nicholas J Hines, Chaitanya A Gadre, Juan Carlos Idrobo, Eungkyu Lee, Karl D Hobart, Mark S Goorsky, Xiaoqing Pan, Tengfei Luo, Samuel Graham
Nat. Commun., 2021, 12, 6901.
DOI: 10.1038/s41467-021-27250-3

Artificial intelligence model for efficient simulation of monatomic phase change material antimony
Mengchao Shi, Junhua Li, Ming Tao, Xin Zhang, Jie Liu
Materials Science in Semiconductor Processing, 2021, 136, 106146.
DOI: 10.1016/j.mssp.2021.106146

Molecular dynamics simulation of metallic Al-Ce liquids using a neural network machine learning interatomic potential
L Tang, K M Ho, C Z Wang
J. Chem. Phys., 2021, 155, 194503.
DOI: 10.1063/5.0066061
Choosing the right molecular machine learning potential
Max Pinheiro Jr, Fuchun Ge, Nicolas Ferr'e, Pavlo O Dral, Mario Barbatti
Chem. Sci., 2021, 12, 14396–14413.
DOI: 10.1039/d1sc03564a

Atomic structure of liquid refractory Nb5Si3 intermetallic compound alloy based upon deep neural network potential
Q. Wang, B. Zhai, H. P. Wang, B. Wei
Journal of Applied Physics, 2021, 130, 185103.
DOI: 10.1063/5.0067157

Azo(xy) vs Aniline Selectivity in Catalytic Nitroarene Reduction by Intermetallics: Experiments and Simulations
Carena L. Daniels, Da-Jiang Liu, Marquix A. S. Adamson, Megan Knobeloch, Javier Vela
J. Phys. Chem. C, 2021, 125 (44), 24440-24450.
DOI: 10.1021/acs.jpcc.1c08569

Resolving the Structural Debate for the Hydrated Excess Proton in Water
Paul B Calio, Chenghan Li, Gregory A Voth
J. Am. Chem. Soc., 2021, 143, 18672–18683.
DOI: 10.1021/jacs.1c08552
Deep-learning potential method to simulate shear viscosity of liquid aluminum at high temperature and high pressure by molecular dynamics
Yuqing Cheng, Han Wang, Shuaichuang Wang, Xingyu Gao, Qiong Li, Jun Fang, Hongzhou Song, Weidong Chu, Gongmu Zhang, Haifeng Song, Haifeng Liu
AIP Advances, 2021, 11, 15043.
DOI: 10.1063/5.0036298
Gold Segregation Improves Electrocatalytic Activity of Icosahedron Au@Pt Nanocluster: Insights from Machine Learning
Dingming Chen, Zhuangzhuang Lai, Jiawei Zhang, Jianfu Chen, Peijun Hu, Haifeng Wang
Chin. J. Chem., 2021, 39, 3029–3036.
DOI: 10.1002/cjoc.202100352
Condensed Phase Water Molecular Multipole Moments from Deep Neural Network Models Trained on Ab Initio Simulation Data
Yu Shi, Carrie C Doyle, Thomas L Beck
J. Phys. Chem. Lett., 2021, 12, 10310–10317.
DOI: 10.1021/acs.jpclett.1c02328
Learning intermolecular forces at liquid-vapor interfaces
Samuel P Niblett, Mirza Galib, David T Limmer
J. Chem. Phys., 2021, 155, 164101.
DOI: 10.1063/5.0067565
Modeling Liquid Water by Climbing up Jacob\textquoterights Ladder in Density Functional Theory Facilitated by Using Deep Neural Network Potentials
Chunyi Zhang, Fujie Tang, Mohan Chen, Jianhang Xu, Linfeng Zhang, Diana Y Qiu, John P Perdew, Michael L Klein, Xifan Wu
J. Phys. Chem. B, 2021, 125, 11444–11456.
DOI: 10.1021/acs.jpcb.1c03884
Deep Density: Circumventing the Kohn-Sham equations via symmetry preserving neural networks
Leonardo Zepeda-N'u\~nez, Yixiao Chen, Jiefu Zhang, Weile Jia, Linfeng Zhang, Lin Lin
Journal of Computational Physics, 2021, 443, 110523.
DOI: 10.1016/j.jcp.2021.110523
First-principles materials simulation and design for alkali and alkaline metal ion batteries accelerated by machine learning
Lujie Jin, Yujin Ji, Hongshuai Wang, Lifeng Ding, Youyong Li
Phys. Chem. Chem. Phys., 2021, 23, 21470–21483.
DOI: 10.1039/d1cp02963k
Local structure elucidation and properties prediction on KCl-CaCl2 molten salt: A deep potential molecular dynamics study
Min Bu, Wenshuo Liang, Guimin Lu, Jianguo Yu
Solar Energy Materials and Solar Cells, 2021, 232, 111346.
DOI: 10.1016/j.solmat.2021.111346
Using Neural Network Force Fields to Ascertain the Quality of Ab Initio Simulations of Liquid Water
Alberto Torres, Luana S Pedroza, Marivi Fernandez-Serra, Alexandre R Rocha
J. Phys. Chem. B, 2021, 125, 10772–10778.
DOI: 10.1021/acs.jpcb.1c04372
Thermal Conductivity of Silicate Liquid Determined by Machine Learning Potentials
Jie Deng, Lars Stixrude
Geophys Res Lett, 2021, 48, e2021GL093806.
DOI: 10.1029/2021GL093806
Ab initio validation on the connection between atomistic and hydrodynamic description to unravel the ion dynamics of warm dense matter
Qiyu Zeng, Xiaoxiang Yu, Yunpeng Yao, Tianyu Gao, Bo Chen, Shen Zhang, Dongdong Kang, Han Wang, Jiayu Dai
Phys. Rev. Research, 2021, 3, 33116.
DOI: 10.1103/PhysRevResearch.3.033116
Liquid-Liquid Critical Point in Phosphorus
Manyi Yang, Tarak Karmakar, Michele Parrinello
Phys. Rev. Lett., 2021, 127, 80603.
DOI: 10.1103/PhysRevLett.127.080603
Robust, Multi-Length-Scale, Machine Learning Potential for Ag–Au Bimetallic Alloys from Clusters to Bulk Materials
Christopher M. Andolina, Marta Bon, Daniele Passerone, Wissam A. Saidi
J. Phys. Chem. C, 2021, 125 (31), 17438-17447.
DOI: 10.1021/acs.jpcc.1c04403
Improved Al-Mg alloy surface segregation predictions with a machine learning atomistic potential
Md Sabbir Akhanda, S Emad Rezaei, Keivan Esfarjani, Sergiy Krylyuk, Albert V Davydov, Mona Zebarjadi
Phys. Rev. Mater., 2021, 5, 83804.
DOI: 10.1103/PhysRevMaterials.5.083804
Anomalous Behavior of Viscosity and Electrical Conductivity of MgSiO 3 Melt at Mantle Conditions
Haiyang Luo, Bijaya B. Karki, Dipta B. Ghosh, Huiming Bao
Geophys Res Lett, 2021, 48.
DOI: 10.1029/2021GL093573
Deep neural network potentials for diffusional lithium isotope fractionation in silicate melts
Haiyang Luo, Bijaya B. Karki, Dipta B. Ghosh, Huiming Bao
Geochimica et Cosmochimica Acta, 2021, 303, 38–50.
DOI: 10.1016/j.gca.2021.03.031
Phase Diagram of a Deep Potential Water Model
Linfeng Zhang, Han Wang, Roberto Car, Weinan E
Phys. Rev. Lett., 2021, 126, 236001.
DOI: 10.1103/PhysRevLett.126.236001
Theoretical prediction on the local structure and transport properties of molten alkali chlorides by deep potentials
Wenshuo Liang, Guimin Lu, Jianguo Yu
Journal of Materials Science & Technology, 2021, 75, 78-85.
DOI: 10.1016/j.jmst.2020.09.040
The thermoelectric performance of new structure SnSe studied by quotient graph and deep learning potential
D. Guo, C. Li, K. Li, B. Shao, D. Chen, Y. Ma, J. Sun, X. Cao, W. Zeng, X. Chang
Materials Today Energy, 2021, 20, 100665.
DOI: 10.1016/j.mtener.2021.100665
Phase Equilibrium of Water with Hexagonal and Cubic Ice Using the SCAN Functional
Pablo M Piaggi, Athanassios Z Panagiotopoulos, Pablo G Debenedetti, Roberto Car
J. Chem. Theory Comput., 2021, 17, 3065–3077.
DOI: 10.1021/acs.jctc.1c00041
Temperature Dependent Thermal and Elastic Properties of High Entropy (Ti0.2Zr0.2Hf0.2Nb0.2Ta0.2)B2: Molecular Dynamics Simulation by Deep Learning Potential
Fu-Zhi Dai, Yinjie Sun, Bo Wen, Huimin Xiang, Yanchun Zhou
Journal of Materials Science & Technology, 2021, 72, 8-15.
DOI: 10.1016/j.jmst.2020.07.014
Theoretical prediction on the redox potentials of rare-earth ions by deep potentials
Jia Zhao, Wenshuo Liang, Guimin Lu
Ionics, 2021, 27, 2079–2088.
DOI: 10.1007/s11581-021-03988-0
Accurate Deep Potential model for the Al-Cu-Mg alloy in the full concentration space*
Wanrun Jiang, Yuzhi Zhang, Linfeng Zhang, Han Wang
Chinese Phys. B, 2021, 30, 50706.
DOI: 10.1088/1674-1056/abf134
Anharmonic Raman spectra simulation of crystals from deep neural networks
Honghui Shang, Haidi Wang
AIP Advances, 2021, 11, 35105.
DOI: 10.1063/5.0040190
Thermal transport by electrons and ions in warm dense aluminum: A combined density functional theory and deep potential study
Qianrui Liu, Junyi Li, Mohan Chen
Matter and Radiation at Extremes, 2021, 6 (2), 026902.
DOI: 10.1063/5.0030123
Crystallization of the P3Sn4 Phase upon Cooling P2Sn5 Liquid by Molecular Dynamics Simulation Using a Machine Learning Interatomic Potential
Chao Zhang, Yang Sun, Hai-Di Wang, Feng Zhang, Tong-Qi Wen, Kai-Ming Ho, Cai-Zhuang Wang
J. Phys. Chem. C, 2021, 125 (5), 3127-3133.
DOI: 10.1021/acs.jpcc.0c08873
Enhancing the formation of ionic defects to study the ice Ih/XI transition with molecular dynamics simulations
Pablo M. Piaggi, Roberto Car
Molecular Physics, 2021, 119.
DOI: 10.1080/00268976.2021.1916634
Static and Dynamic Correlations in Water: Comparison of Classical Ab Initio Molecular Dynamics at Elevated Temperature with Path Integral Simulations at Ambient Temperature
Chenghan Li, Francesco Paesani, Gregory A Voth
J. Chem. Theory Comput., 2022, 18, 2124–2131.
DOI: 10.1021/acs.jctc.1c01223
Molecular dynamics simulations of lanthanum chloride by deep learning potential
Taixi Feng, Jia Zhao, Wenshuo Liang, Guimin Lu
Computational Materials Science, 2021, 111014.
DOI: 10.1016/j.commatsci.2021.111014
Diffusional fractionation of helium isotopes in silicate melts
H. Luo, B.B. Karki, D.B. Ghosh, H. Bao
Geochem. Persp. Let., 2021, 19–22.
DOI: 10.7185/geochemlet.2128
Short- and medium-range orders in Al90Tb10 glass and their relation to the structures of competing crystalline phases
L. Tang, Z.J. Yang, T.Q. Wen, K.M. Ho, M.J. Kramer, C.Z. Wang
Acta Materialia, 2021, 204, 116513.
DOI: 10.1016/j.actamat.2020.116513
A DFT accurate machine learning description of molten ZnCl2 and its mixtures: 2. Potential development and properties prediction of ZnCl2-NaCl-KCl ternary salt for CSP
Gechuanqi Pan, Jing Ding, Yunfei Du, Duu-Jong Lee, Yutong Lu
Computational Materials Science, 2021, 187, 110055.
DOI: 10.1016/j.commatsci.2020.110055
Deep learning of accurate force field of ferroelectric HfO2
Jing Wu, Yuzhi Zhang, Linfeng Zhang, Shi Liu
Phys. Rev. B, 2021, 103, 24108.
DOI: 10.1103/PhysRevB.103.024108
Machine-Learning-Driven Simulations on Microstructure and Thermophysical Properties of MgCl2-KCl Eutectic
Wenshuo Liang, Guimin Lu, Jianguo Yu
ACS Appl. Mater. Interfaces, 2021, 13, 4034–4042.
DOI: 10.1021/acsami.0c20665
Theoretical study of Na+ transport in the solid-state electrolyte Na3OBr based on deep potential molecular dynamics
Han-Xiao Li, Xu-Yuan Zhou, Yue-Chao Wang, Hong Jiang
Inorg. Chem. Front., 2021, 8, 425–432.
DOI: 10.1039/D0QI00921K
When do short-range atomistic machine-learning models fall short?
Shuwen Yue, Maria Carolina Muniz, Marcos F Calegari Andrade, Linfeng Zhang, Roberto Car, Athanassios Z Panagiotopoulos
J. Chem. Phys., 2021, 154, 34111.
DOI: 10.1063/5.0031215
2020
Free energy of proton transfer at the water-TiO2 interface from ab initio deep potential molecular dynamics
Marcos F Calegari Andrade, Hsin-Yu Ko, Linfeng Zhang, Roberto Car, Annabella Selloni
Chem. Sci., 2020, 11, 2335–2341.
DOI: 10.1039/C9SC05116C

Theoretical prediction on thermal and mechanical properties of high entropy (Zr0.2Hf0.2Ti0.2Nb0.2Ta0.2)C by deep learning potential
Fu-Zhi Dai, Bo Wen, Yinjie Sun, Huimin Xiang, Yanchun Zhou
Journal of Materials Science & Technology, 2020, 43, 168–174.
DOI: 10.1016/j.jmst.2020.01.005

A deep neural network interatomic potential for studying thermal conductivity of $\beta$-Ga2O3
Ruiyang Li, Zeyu Liu, Andrew Rohskopf, Kiarash Gordiz, Asegun Henry, Eungkyu Lee, Tengfei Luo
Appl. Phys. Lett., 2020, 117, 152102.
DOI: 10.1063/5.0025051

Structure and dynamics of warm dense aluminum: a molecular dynamics study with density functional theory and deep potential
Qianrui Liu, Denghui Lu, Mohan Chen
J. Phys. Condens. Matter, 2020, 32, 144002.
DOI: 10.1088/1361-648X/ab5890
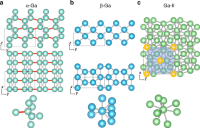
Ab initio phase diagram and nucleation of gallium
Haiyang Niu, Luigi Bonati, Pablo M Piaggi, Michele Parrinello
Nat. Commun., 2020, 11, 2654.
DOI: 10.1038/s41467-020-16372-9
Raman spectrum and polarizability of liquid water from deep neural networks
Grace M Sommers, Marcos F Calegari Andrade, Linfeng Zhang, Han Wang, Roberto Car
Phys. Chem. Chem. Phys., 2020, 22, 10592–10602.
DOI: 10.1039/D0CP01893G

A machine learning based deep potential for seeking the low-lying candidates of Al clusters
P Tuo, X B Ye, B C Pan
J. Chem. Phys., 2020, 152, 114105.
DOI: 10.1063/5.0001491
Data-driven coarse-grained modeling of polymers in solution with structural and dynamic properties conserved
Shu Wang, Zhan Ma, Wenxiao Pan
Soft Matter, 2020, 16, 8330–8344.
DOI: 10.1039/D0SM01019G
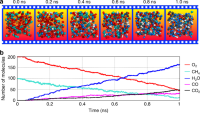
Complex reaction processes in combustion unraveled by neural network- based molecular dynamics simulation
Jinzhe Zeng, Liqun Cao, Mingyuan Xu, Tong Zhu, John Z H Zhang
Nat. Commun., 2020, 11, 5713.
DOI: 10.1038/s41467-020-19497-z
Deep neural network for the dielectric response of insulators
Linfeng Zhang, Mohan Chen, Xifan Wu, Han Wang, Weinan E, Roberto Car
Phys. Rev. B, 2020, 102, 41121.
DOI: 10.1103/PhysRevB.102.041121

DP-GEN: A concurrent learning platform for the generation of reliable deep learning based potential energy models
Yuzhi Zhang, Haidi Wang, Weijie Chen, Jinzhe Zeng, Linfeng Zhang, Han Wang, Weinan E
Computer Physics Communications, 2020, 253, 107206.
DOI: 10.1016/j.cpc.2020.107206
Isotope effects in molecular structures and electronic properties of liquid water via deep potential molecular dynamics based on the SCAN functional
Jianhang Xu, Chunyi Zhang, Linfeng Zhang, Mohan Chen, Biswajit Santra, Xifan Wu
Phys. Rev. B, 2020, 102, 214113.
DOI: 10.1103/PhysRevB.102.214113

Hydrogen Dynamics in Supercritical Water Probed by Neutron Scattering and Computer Simulations
Carla Andreani, Giovanni Romanelli, Alexandra Parmentier, Roberto Senesi, Alexander I Kolesnikov, Hsin-Yu Ko, Marcos F Calegari Andrade, Roberto Car
J. Phys. Chem. Lett., 2020, 11, 9461–9467.
DOI: 10.1021/acs.jpclett.0c02547

A unified deep neural network potential capable of predicting thermal conductivity of silicon in different phases
R. Li, E. Lee, T. Luo
Materials Today Physics, 2020, 12, 100181.
DOI: 10.1016/j.mtphys.2020.100181

Combining the Fragmentation Approach and Neural Network Potential Energy Surfaces of Fragments for Accurate Calculation of Protein Energy
Zhilong Wang, Yanqiang Han, Jinjin Li, Xiao He
J. Phys. Chem. B, 2020, 124, 3027–3035.
DOI: 10.1021/acs.jpcb.0c01370

A Deep-Learning Potential for Crystalline and Amorphous Li-Si Alloys
Nan Xu, Yao Shi, Yi He, Qing Shao
J. Phys. Chem. C, 2020, 124, 16278–16288.
DOI: 10.1021/acs.jpcc.0c03333
Development of interatomic potential for Al-Tb alloys using a deep neural network learning method
L Tang, Z J Yang, T Q Wen, K M Ho, M J Kramer, C Z Wang
Phys. Chem. Chem. Phys., 2020, 22, 18467–18479.
DOI: 10.1039/D0CP01689F
Isotope effects in x-ray absorption spectra of liquid water
Chunyi Zhang, Linfeng Zhang, Jianhang Xu, Fujie Tang, Biswajit Santra, Xifan Wu
Phys. Rev. B, 2020, 102, 115155.
DOI: 10.1103/PhysRevB.102.115155

Warm dense matter simulation via electron temperature dependent deep potential molecular dynamics
Yuzhi Zhang, Chang Gao, Qianrui Liu, Linfeng Zhang, Han Wang, Mohan Chen
Physics of Plasmas, 2020, 27, 122704.
DOI: 10.1063/5.0023265

Molecular Dynamics Simulations of Molten Magnesium Chloride Using Machine-Learning-Based Deep Potential
Wenshuo Liang, Guimin Lu, Jianguo Yu
Adv. Theory Simul., 2020, 3, 2000180.
DOI: 10.1002/adts.202000180

Grain boundary strengthening in ZrB2 by segregation of W: Atomistic simulations with deep learning potential
Fu-Zhi Dai, Bo Wen, Huimin Xiang, Yanchun Zhou
Journal of the European Ceramic Society, 2020, 40, 5029–5036.
DOI: 10.1016/j.jeurceramsoc.2020.06.007
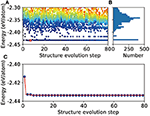
Crystal Structure Prediction of Binary Alloys via Deep Potential
Haidi Wang, Yuzhi Zhang, Linfeng Zhang, Han Wang
Front. Chem., 2020, 8, 589795.
DOI: 10.3389/fchem.2020.589795
Deep machine learning interatomic potential for liquid silica
I A Balyakin, S V Rempel, R E Ryltsev, A A Rempel
Phys. Rev. E, 2020, 102, 52125.
DOI: 10.1103/PhysRevE.102.052125
Structure of disordered TiO2 phases from ab initio based deep neural network simulations
Marcos F. Calegari Andrade, Annabella Selloni
Phys. Rev. Materials, 2020, 4, 113803.
DOI: 10.1103/PhysRevMaterials.4.113803

Signatures of a liquid-liquid transition in an ab initio deep neural network model for water
Thomas E Gartner 3rd, Linfeng Zhang, Pablo M Piaggi, Roberto Car, Athanassios Z Panagiotopoulos, Pablo G Debenedetti
Proc. Natl. Acad. Sci. U. S. A., 2020, 117, 26040–26046.
DOI: 10.1073/pnas.2015440117
2019
Active learning of uniformly accurate interatomic potentials for materials simulation
Linfeng Zhang, De-Ye Lin, Han Wang, Roberto Car, Weinan E
Phys. Rev. Materials, 2019, 3, 23804.
DOI: 10.1103/PhysRevMaterials.3.023804

Isotope effects in liquid water via deep potential molecular dynamics
Hsin-Yu Ko, Linfeng Zhang, Biswajit Santra, Han Wang, Weinan E, Robert A. DiStasio Jr, Roberto Car
Molecular Physics, 2019, 117, 3269–3281.
DOI: 10.1080/00268976.2019.1652366
Development of a deep machine learning interatomic potential for metalloid-containing Pd-Si compounds
Tongqi Wen, Cai-Zhuang Wang, M. J. Kramer, Yang Sun, Beilin Ye, Haidi Wang, Xueyuan Liu, Chao Zhang, Feng Zhang, Kai-Ming Ho, Nan Wang
Phys. Rev. B, 2019, 100, 174101.
DOI: 10.1103/PhysRevB.100.174101

Deep learning inter-atomic potential model for accurate irradiation damage simulations
Hao Wang, Xun Guo, Linfeng Zhang, Han Wang, Jianming Xue
Appl. Phys. Lett., 2019, 114, 244101.
DOI: 10.1063/1.5098061
2018
Silicon Liquid Structure and Crystal Nucleation from Ab~Initio Deep Metadynamics
Luigi Bonati, Michele Parrinello
Phys. Rev. Lett., 2018, 121, 265701.
DOI: 10.1103/PhysRevLett.121.265701

Deep Learning for Nonadiabatic Excited-State Dynamics
Wen-Kai Chen, Xiang-Yang Liu, Wei-Hai Fang, Pavlo O Dral, Ganglong Cui
J. Phys. Chem. Lett., 2018, 9, 6702–6708.
DOI: 10.1021/acs.jpclett.8b03026

Adaptive coupling of a deep neural network potential to a classical force field
Linfeng Zhang, Han Wang, Weinan E
J. Chem. Phys., 2018, 149, 154107.
DOI: 10.1063/1.5042714

DeePCG: Constructing coarse-grained models via deep neural networks
Linfeng Zhang, Jiequn Han, Han Wang, Roberto Car, Weinan E
J. Chem. Phys., 2018, 149, 34101.
DOI: 10.1063/1.5027645

DeePMD-kit: A deep learning package for many-body potential energy representation and molecular dynamics
Han Wang, Linfeng Zhang, Jiequn Han, Weinan E
Computer Physics Communications, 2018, 228, 178–184.
DOI: 10.1016/j.cpc.2018.03.016
Deep Potential Molecular Dynamics: A Scalable Model with the Accuracy of Quantum Mechanics
Linfeng Zhang, Jiequn Han, Han Wang, Roberto Car, Weinan E
Phys. Rev. Lett., 2018, 120, 143001.
DOI: 10.1103/PhysRevLett.120.143001